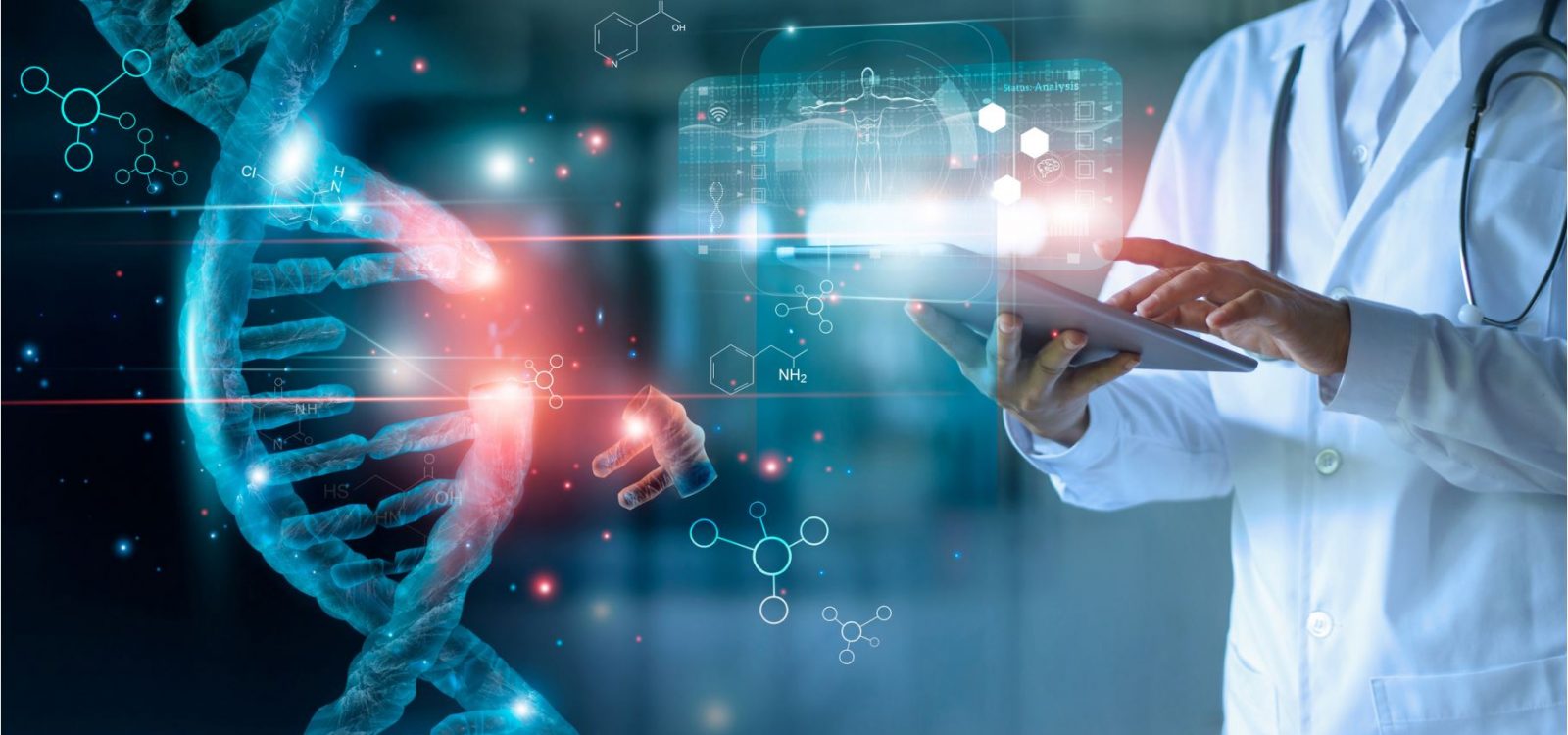
The far future of healthcare – FOHS Plenary 2 report
The future of decision-making (in healthcare and broader contexts) relies on making data usable to support the best choices. An important consideration for the future of our healthcare is that this data has to reflect the population it is being used on.
Data is the new natural resource
It isn’t always the case that data is being applied to the right populations. Global health recommendations are often based on data from those with better healthcare
access in wealthier countries, since this is where sources of data (electronic medical records) are most well established, Adam Dunn, Associate Professor, Centre for Health Informatics, Macquarie University, explained.
“Clinical trials are about as representative of the population as the ninth episode of the Bachelorette”
Stefan Harrer of IBM Research also spoke to the development of AI and importance of data, beginning his talk with introducing data as “the new natural resource’.
Data can be sourced from everywhere from social media and apps (including wearable devices) to clinical data and medical imaging.
Machine learning and artificial intelligence
Despite all the hype around Artificial intelligence (AI), Stefan highlighted how the technology is it is still in its early days. We’re just emerging from narrow AI (primarily proof of concepts) into more ‘Broad AI’ that is disruptive and pervasive.
Because of the growing ability of AI to influence our lives, it is important to consider the ethics of AI development. Stefan offered five needs that we should require of AI development:
-
Accountability
-
Value alignment – just because you can build it, doesn’t mean you should
-
Explainability – being able to understand the process is important when you’re impacting people’s lives so materially.
-
Fairness – to minimise bias and promote inclusion
-
User data rights
One ongoing application is in pharmaceutical drug makers’ investment in new drugs. Investments in drug development are very large, nearly $1 billion over 10-15 years for a new drug. The process is incredibly inefficient and has high risks since clinical trials can show limited effects and fail. There are various causes, but many can be helped with AI to make the process more efficient.
AI can help with cohort composition, patient recruitment and patient monitoring – ultimately resulting in optimised cohort compositions, with lower dropout rates and improved patient adherence. This translates to less expensive trials and increased likelihood of success.
Beyond the general improvements to current operations, Adam Dunn poses two questions:
- how do we make sure machine learning algorithms and the data that they are trained on do not inadvertently increase inequity?
- can machine learning instead help us identify, reach and support under-served populations?
The answer depends on our ability to source data. While traditional sources of health data are based on interactions with the healthcare system (and subject to a biased sample), mobile phones offer a solution to supporting and serving a broader population.
Many places in the world have “access to mobile phones is higher than access to running water” and mobile applications to healthcare are growing. They include
-
Identifying potential depressive episodes from the rate at which a person types messages on their phone keyboards
-
Skin cancer classification using deep learning on images taken from cell phone cameras
-
Automatic diagnosis of diabetic retinopathy
But rolling out these applications needs to be done cautiously – there was an instance of an application designed to help triage patients into the ER, normal doctors visit or just stay home – that would tell you to stay home with symptoms of a heart attack.
Genomics
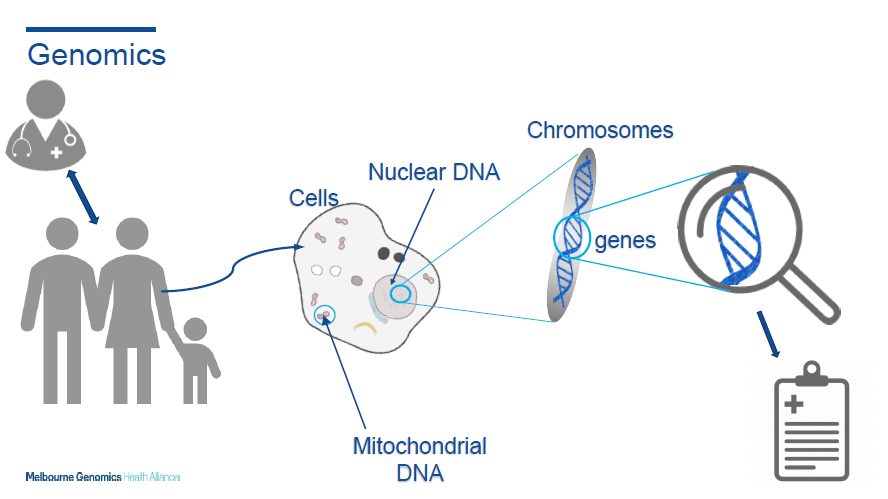
Genomic sequencing traditionally has been expensive and slow. It could take 4-6 months to sequence one gene, with a price tag of several thousand dollars. This has changed in recent years. Today we are able to sequence all ~20,000 genes in potentially as short as 48 hours.
Today, only a subset are currently analysed for the patient specific ‘disease causing variance’. But we’re continuing to learn more about genes that impact future healthcare needs. As this knowledge base develops, we’ll have to address some profound questions like: ‘if I’m being tested for something specific, do I want to know about other abnormalities that might be found?’ What if they’re medically actionable? What if they’re not? How will this impact my use of healthcare services?
“Of patients who receive a genomics diagnostic, 55% of them had a change in care.”
There are clear advantages of introducing genomic sequencing more widely. Elly Lynch, Senior Genetic Counsellor, Melbourne Genomics Health Alliance shared the story of Abigail – a baby who was healthy until reaching about 8 months when she started having seizures. She stopped developing and had many intensive healthcare interventions. None of these tests lead to any results.
Abigail was recruited into the Melbourne Genomics study to look her genes rapidly. After looking through her genes, the sequencing identified that Abigail couldn’t transport vitamin B2 around her body. A simple daily dose of this vitamin stopped the seizures and saved her life. This also saved the healthcare system a significant cost savings of $500,000.
The public healthcare system has identified that genomic sequencing can improve the future of healthcare diagnostics, if properly embedded into the healthcare system.
Read moreSee a summary of other Plenary sessions written by Brendan Pon, Lulu Wang, Nora Lam and Zachary Tirrell |
CPD: Actuaries Institute Members can claim two CPD points for every hour of reading articles on Actuaries Digital.