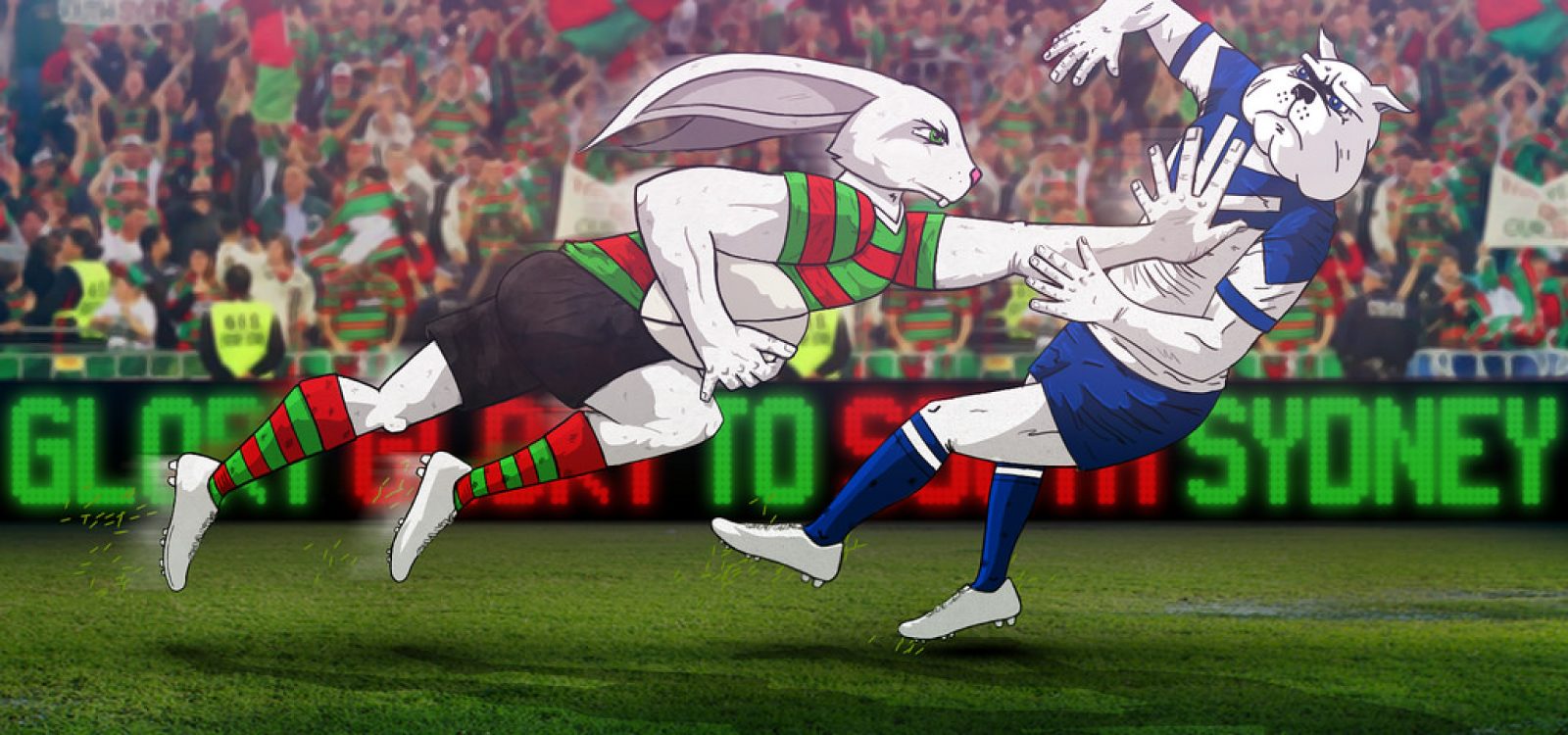
Beat the Geek: Actuarial tips on AFL and NRL this season
Taylor Fry is celebrating the 10th year of its ‘Beat the Geek’ AFL and NRL tipping competition which pits tipsters skills against the expert modelling power of an actuarial and analytics firm. Here, Graham Taylor lets us in on common tipster habits, what works and of course, who will win the 2017 premierships!
Q How would you describe ‘Beat the Geek’ competition?
A Our “Beat the Geek” competition was conceived in 2008 by a former colleague, James Sullivan, to engage and entertain our clients. We initially developed three models (two GLMs and a Boosted decision tree model) to tip the winners of AFL games and invited our clients to compete against these models, with prizes for the best tipsters to encourage take-up. We added a NRL competition in 2010 and this year is the 10th anniversary of our comps.
Q What have you learned about how people tip, what works and why?
A There is a wide spectrum of tipsters (we are all just part of a distribution). Three common approaches include:
- Slow and steady – wins the race – these people generally tip favourites and often do well.
- All or nothing – these tipsters will take a few chances each round. They often win the weekly prize (most winners picked correctly in a round) although only very rarely win our comps – there’s typically a strong inverse correlation between weekly round winners and comp winners.
- Hit and hope – employ more of a random approach to tipping. At least they make everyone else look good.
Q Can you let us in on how the models work?
A As already mentioned we built two Generalised linear models (GLMs) and a Boosted decision tree model. Due to the lack of data (and some laziness in collecting additional data) the GLMs are fairly simple. The tips can be viewed as ‘odds-plus’ models, since betting odds are by far the most useful predictor –some of our tipsters such as Michael Price (AMP) had already worked that out themselves. The odds have tended to carry small biases over time, so the additional factors we model (even a simple home ground factor) can improve the signal slightly. An interesting aspect of fitting the GLMs is that limited amount of data requires significant care in the setup choice of factors. This is quite different from some of our client models where big datasets allow everything to be tested together. The boosted decision tree model builds a ‘black box’ model, which is harder to interpret although it does produce a rank of variable significance.
Q How have the models fared over the years?
A I would give a scorecard of 7 to 8 out of 10. The models have always finished in the top half of our client competition, they have won the comps twice (one year finishing 83rd out of 108,000 on the wider footytips.com.au website). The NRL models have tended to finish higher on the leaderboard than the AFL models. Over the years, the more manual GLM models have done marginally better than the boosted decision tree model (hopefully my manager is reading this – there’s still some hope we don’t get replaced by robots).
Q Can money be made from modelling sports data?
A Yes (David Walsh seems to have done OK). A few years ago a derivatives trader and professional gambler approached us about supplying him with certain information from the models. We had noticed that the models were particular good at predicting a certain aspect of a match and, on the limited amount of data, reduced the bookies’ typical margin from around 5% in their favour (that is, over the long run you get 95% back on a bet) to moderately in our favour (slightly over 100%). We did consider the business case – the size of the bets required, the relatively small number of AFL and NRL matches and the non zero probability of ruin meant that we decided against pursuing it. One related finding is that fixed odds betting agencies do not always get it right. A number of years ago bookies typically offered the same odds for a half-time/full-time draw in AFL and NRL irrespective of the head to head odds – this is clearly wrong, since well-matched teams are much more likely to tie. They have since amended this, mainly by reducing odds on games expected to be close.
Having said all that almost every man and his dog has tried to beat the odds and the vast majority have failed.
Q Who should we back this AFL/NRL season (premiere predictions?) and why?
A GWS in the AFL and Melbourne Storm in the NRL, although boringly these are the same as the betting market favourites. If you are taking part in a tipping comp this year then my recommended strategy would be to tip with the bookies’ favourites except for 4 or 5 times over the season – if you get those correct you will have a good chance of winning the comp!
Header Image by Scott Nolan.
CPD: Actuaries Institute Members can claim two CPD points for every hour of reading articles on Actuaries Digital.