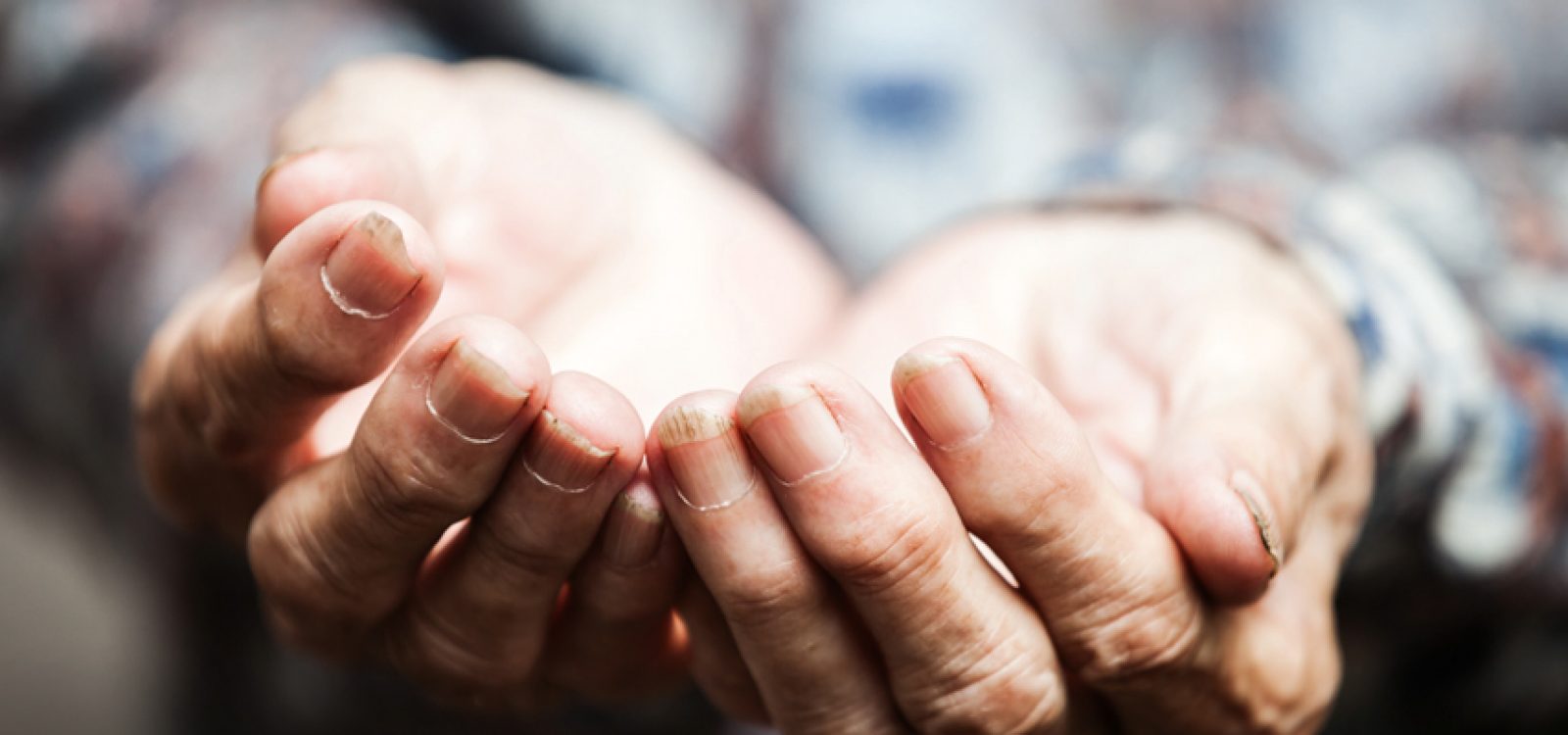
Latest Developments in Mortality Projection Methods
Mortality rates in Australia and New Zealand have declined over time, and there is no sign of this trend abating –creating significant implications for pricing and funding scenarios into the future.
RISING LONGEVITY
Increasing life expectancy poses a significant challenge to many insurers, pension plan sponsors, and governments. The major concern is the so-called longevity risk, which is the risk of higher than expected payouts due to mortality improvement.
The graphs to the right show that the mortality levels of Australia and New Zealand have been declining over time. In fact, life expectancy of these two countries has increased by around three months for every year in recent decades, and there is no sign of this upward trend ending in the foreseeable future.
For pricing, reserving, and funding purposes, it is of utmost importance to study carefully the past patterns of mortality movements and to make a proper assessment of future trends.
Figure 1: Death rates in Australia (log scale)
Figure 2: Death rates in New Zealand (log scale)
GENERAL APPROACHES
Broadly speaking, there are three approaches for forecasting mortality – expectation, explanation, and extrapolation. Each approach has its own advantages and disadvantages, as outlined in the table below, but extrapolation remains the most prevalent approach. This approach is categorised by assuming certain past trends will continue in the future.
Table 1: three general approaches of forecasting mortality
APPROACH |
INPUTS |
OUTPUTS |
ADVANTAGES |
DISADVANTAGES |
Expectation |
• individual expectations • expert opinions |
• aggregate measures |
• can incorporate structural changes and unusual incidents |
• too pessimistic • limited by views of current technology • no specific details |
Explanation |
|
• detailed relationships between variables |
• allow for precise mechanism underlying mortality changes |
|
Extrapolation |
• past mortality trends • mortality models |
• model forecasts |
|
|
Some critics argue that this approach does not allow for the underlying mechanisms which dictate how different variables change over time. However, projecting past, relevant trends still represents a solid benchmark for further analysis.
The projection results can be adjusted based on other related information, experts’ opinions, and professional judgement. Moreover, sensitivity testing can be carried out to examine the impact of changing the initial assumptions. Overall, extrapolation serves as a transparent, systematic, and statistically sound way to estimate future mortality and is very useful in various kinds of actuarial calculations and population projection.
EXTRAPOLATION METHODS
There are discrete-time and continuous-time mortality projection models. The former is defined for data collected at regular intervals, whereas the latter involves stochastic processes and complex mathematics. Because mortality data is usually summarised yearly, most work thus far uses discrete-time models.
The simplest discrete-time approach is probably calculating the average rate of decline (per year) of the death rate at each age or age group. However, this approach may produce irregular age patterns as the death rate at each age is projected to change at its own rate. This approach does not give a representative index which could reflect the overall mortality level.
Besides the simple approach above, there are two main categories of discrete-time models that have been thoroughly tested and discussed in the literature over the past 20 years.
The first category is the Lee-Carter (LC) model and its various extensions, which have the following basic format:
log death rate = age schedule +
age-specific sensitivity x mortality index
Numerous studies have demonstrated that the mortality index declines fairly linearly over time after around 1970 for many countries’ data (seen in the following figure), suggesting that one may project future mortality by simply extrapolating the linear trend.
The LC model has the advantage that its mortality index is easy to interpret as an indicator of overall mortality levels across time, but the use of a single index and unsmoothed age-sensitivity factors may lead to irregular forecasts.
Figure 3: Lee-Carter mortality index for Australia
The other category is projecting the parameters of a mortality curve. Some notable examples are the Gompertz, Weibull, Heligman-Pollard, and logistic models.
In particular, the Cairns-Blake-Dowd (CBD) model, which has been covered in many recent studies, is a reduced form of the Heligman- Pollard curve. The specification is:
logit death rate = intercept + slope x (age – mean age)
The intercept and slope are treated as mortality indices, which are projected by a time series model. All these models ensure a reasonable mortality schedule in the projection. Nevertheless, if the chosen mortality curve has too many parameters, the multivariate modelling process can be very tedious. One solution is to focus on a certain age range, e.g. the CBD model deals with ages 60 to 90 and has only two parameters.
MULTIPLE POPULATIONS
Most of the current literature has either projected each population separately (by sex, country etc.) or the entire combined population as a whole. Less attention has been paid to modelling two or more related populations jointly.
This joint modelling is particularly important when either:
- it is necessary to make sure that projected death rates of different populations do not diverge;
- the data being studied is sparse and reference has to be made to a larger population; or
- the portfolio to be hedged and the hedging instrument have different underlying populations and basis risk needs to be assessed.
In broad terms, there are three types of multi-population models.
The first type is to fit a single-population model to each population separately and then to model the dependence between the multiple mortality indices in some way. This method is straightforward but it usually generates divergent projected values.
The second type is to incorporate a common factor for all populations in aggregate and also specific factors for each population. The key advantage of this type of model is that the resulting projections are ‘coherent’ and do not diverge.
The final type of joint modelling specifies the ratio of death rates between two populations as a function of age and period factors. This method also produces coherent projections.
SIMULATION METHODS
In addition to the best (central) estimate, it is also of practical interest to obtain a probability distribution which describes how actual outcomes may differ from expected. This is especially so under the current regulatory environment, which has become more risk-based. For example, QIS5 Technical Specifications for Solvency II states that “the nature of the risks underlying the insurance contracts could be described by the probability distribution of the future cash flows arising from the contracts”.
Prudential Standard LPS 340 by APRA also stipulates that “in determining the best estimate liability and best estimate assumptions, the life company must have regard to the impact on the liability of the distribution of potential future outcomes”.
One can use this probability distribution to assess certain risk metrics (e.g. standard deviation, variance, Value-at-Risk) in pricing, hedging, reserving, and capital management. Since deriving the probability distribution for most mortality projection models is analytically intractable, simulation methods are needed to generate random samples of future outcomes. In principle, process error (random fluctuations), parameter error (uncertainty in parameter estimation) and model error (model misspecification) should all be taken into account in the simulation process.
In the literature, there are basically six kinds of simulation methods based on the LC model. These include:
- considering the error term of the mortality index only;
- including the estimation error of the drift term;
- simulating the parameters from the multivariate normal distribution;
- bootstrapping the number of deaths from the Poisson distribution;
- bootstrapping the residuals of the fitted model; and
- Bayesian Markov Chain Monte Carlo (MCMC) simulation.
For the first two methods, the programming is fairly straightforward and the computation time is short. Comparatively, the last four methods are much more demanding and time-consuming in general, but they offer a more precise and sophisticated mechanism for integrating different errors.
PRACTICAL USE
Longevity risk is a significant issue facing many insurers and governments. The existing literature provides a rich source of mortality projection methods, which can help practitioners study past mortality changes in more detail and make better forecasts.
Particularly, as insurance regulations evolve to become more risk-based, it is expected that stochastic mortality modelling will grow in importance. For instance, the following figure shows the simulated distribution of the present value of an annuity of $1 per annum for a New Zealand female aged 65 on 1 January 2010, in which the discount rate is assumed to be 6% per annum. The mean, standard deviation, and different percentiles can readily be estimated from the simulated samples.
Figure 4: Simulated distribution of present value of an annuity using New Zealand data and Lee-Carter model
Unsurprisingly, each mortality model or simulation method has its own merits and deficiencies. Therefore, it is essential to have a good understanding of the main assumptions and features of the chosen model, and to consider its appropriateness for the purpose for which it is being used.
Ideally, a practitioner should test different approaches and examine the impact on the calculation results.
Stochastic mortality modelling is gaining popularity in overseas countries such as the US and UK, with more and more insurers attempting to adopt certain advanced methodologies to assess their businesses.
Those institutions that do not try and experiment with these emerging tools at all run the risk of being regarded as obsolete in their valuation methods among their peers. This trend in turn would lead to better data collection, preparation, and analysis, and more theoretically sound techniques to be developed for use in practice.
For those interested in future reading, the literature referenced in this article are:
- Tickle, L. and Booth, H. (2014). The Longevity Prospects of Australian Seniors: An evaluation of forecast method and outcome. Asia Pacific Journal of Risk and Insurance (forthcoming).
- Li J. 2014. A quantitative comparison of simulation strategies for mortality projection. Annals of Actuarial Science (forthcoming).
CPD: Actuaries Institute Members can claim two CPD points for every hour of reading articles on Actuaries Digital.