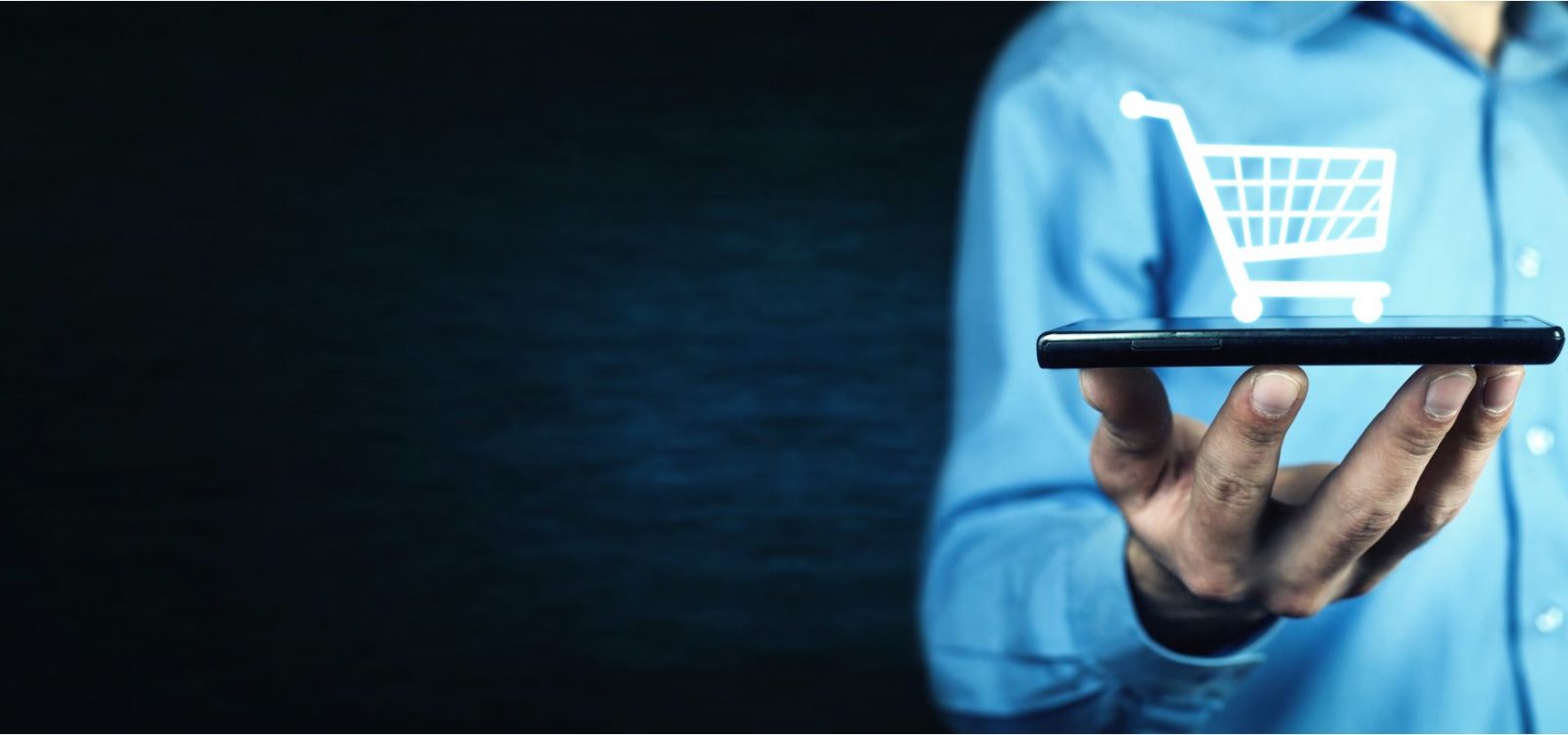
Transform your business with predictive analytics
This article aims to demonstrate the utility of predictive analytics models to the life and annuity spaces and how it can be maximised to better retain and engage customers.
Predictive analytics and artificial intelligence (AI) are one of the most transformative developments in the history of life and annuity products. Early adapters are poised to achieve major strategic advantages.We now see many companies with “Chief Analytics Officers” and there is a recent Academy of Actuaries monograph on big data.
Why is predictive analytics important to the life insurance and annuity spaces? It can help us:
- Improve the dynamics of our business; and
- Reduce variability in our financial statements.
Predictive analytics can enable us to better understand the complex causal relationships that affect the performance of our business, in real time, thereby enabling exponential strategic advantages.. In other words, we have the predictive insights in time to act on them, enabling the business to be proactive rather than reactive.
Before elaborating on this, a word of caution. “All models are wrong, but some are useful” said the famous statistician George E.P. Box. Models for predictive analysis are no exception.
The value of data
In the 1930’s, before the computer age, Eunice Hunton Carter, an Assistant District Attorney in New York, effectively and efficiently used the data available to her – primarily evidence and reports presented by people visiting her office – to build a massive prostitution racketeering case against Lucky Luciano. This case was successfully prosecuted leading to the conviction, imprisonment, and deportation of Luciano, a major organised crime boss. It was the most successful prosection of organised crime up to that time. Data is powerful!
Fast forward to the 21st Century. While browsing an online store like Amazon, we will be told “You might also like …,” “Recommended for you …,” or “Customers who bought this item also bought …” We are consistently surprised and even amazed that the exact product we like or need are displayed. Online shopping giants have effectively mined their data troves and used data science to identify what their customers are likely to need.
Personalisation in life insurance industry
I own a life insurance policy from one of the largest life insurers. The closest they have come to recommending me a product is to send a list of all the products they offer and suggest that I spend time discussing my needs with the producer. I also own automobile and homeowners insurance from one of the largest P&C writers. Every few years they recommend that I buy $100,000 of life insurance. But this is hardly personalised! What will it take for our industry to catch up to the likes of Amazon?
Life insurers are using predictive analytics in certain instances – such as with Accelerated (or Automated) Underwriting (AU), post-level term lapsation and premium setting. However, in other instances (e.g., sales and retention), it would appear that we may not be making as much use of predictive analytics as we could.
Perhaps experts have studied this and determined that additional predictive analytics is unable to help our industry. However, I believe this is simply not true. Suppose that Company A has poor lapse experience and wants to determine what it can do to improve its persistency. They can call everyone who lapses, find out their issues, and try to convince them to reinstate. But, at best, this would be expensive and after the fact. They could call in-force policyholders instead before lapsation happens, but it would be hit or miss on whether they are calling customers at risk, and hence an expensive proposition with dubious results. Worse yet, some policyholders who otherwise would not have lapsed may get the idea from these calls to lapse their policies. So, how can predictive analytics help Company A improve its retention?.
Knowledge is power
Predictive analytics – without human intervention – has demonstrated that some data, such as the premium payment date – previously thought of by many as important only for administrative purposes – can be significant predictors of lapsation risk. Lower and middle income customers who pay their premiums shortly after they receive their paycheck, when they have sufficient funds in their checking account, are more likely to keep their policies in force. Those customers whose premium due dates fall a longer time after they receive their pay checks – by which time they may have spent their most recent paycheck – are more likely to lapse. Armed with this knowledge and other discoveries generated by predictive analytics, insurers and producers can know which policyholders to call and when as well as why these customers are at high risk. The machine makes these connections by itself without anyone needing to know them beforehand.
Using predictive analytics for retention is particularly powerful as the model can be extended to related use cases. Once a predictive model is set up to improve retention, it can be further developed to provide more accurate financials with lower variability. This can be achieved by strengthening the assumption setting process for lapsation.
Strengthening lapse rate assumptions
One big issue in building lapse rate assumptions is the combination of experience from different economic or interest rate environments. For mortality we routinely combine together experience of five years and assume that the year with a particularly harsh winter and a flu epidemic is offset by the year with a particularly mild winter. On the other hand, for lapses this is much more difficult due to the many different combinations of interest rate environments we can have and the fact that they do not necessarily average out.
“How do we use predictive analytics to effectively combine lapse experience of periods in close proximity to each other that have different interest rate environments?”
One fundamental aspect of predictive analytics is feature engineering. Feature engineering uses domain knowledge of the problem being studied (e.g., the setting of lapse assumptions) to create variables that make the algorithms work. These can be tested for significance and incorporated into a model. For example, the magnitude and changes in interest rates can be tested for significance in a lapse model.
Once a sufficiently detailed model is developed, it would then identify the appropriate policyholder segments by which to analyse the lapse experience as well as predict lapse rates for each segment. Segments could have markedly different behaviour, but should be studied only if they have sufficient credibility.
Based on this, the predictive analytics model would use the selected variables to identify the impact that the interest rate environment (as well as other factors) has on lapses, and the model could effectively identify a base lapse rate vector that is independent of the current interest rate environment. This allows separation of some cyclical factors from customer trends.
“We produce more refined policyholder segments that have been newly identified and are using more data and extended study periods to set credible lapse rate assumptions with lower variability.”
The lapse assumptions are more accurate than those produced previously and financial models and results will have lower variability. It also provides a launchpad for reducing lapses in the future. Whether this support has strong incremental effects or exponential strategic advantages depends on the insurer’s implementation.
Automation
To achieve exponential strategic advantages, the insurer would automate the predictive analytics. The automation would enable expeditious analysis of additional potentially predictive factors that arise as well as real time learnings on the impact of behavioural, economic, market and other environmental changes. The insurer can then be proactive in improving policyholder retention and understanding its emerging lapse experience.
Returning to the sales process, let us think about how much valuable information we collect that we do not use. For example, when a policyholder notifies us of a change in address, do we treat it purely as an administrative matter or do we analyse it to see whether the move suggests changed economic or family circumstances and hence an increased need for coverage?
Given that many people simply do not buy what a needs analysis says they should buy, perhaps we can start by letting people know how much coverage others in similar circumstances have. This may not solve the entire gap in life insurance coverage, but it is a message that resonates with customers (as Amazon has demonstrated) and it would be a door opener for us to talk to customers and prospects about their needs.
We in the life and annuity spaces have built our businesses by collecting and effectively analysing huge volumes of data. Let us continue to innovate and use the new tools now available to us to revitalise – and indeed revolutionise – our businesses!
CPD: Actuaries Institute Members can claim two CPD points for every hour of reading articles on Actuaries Digital.