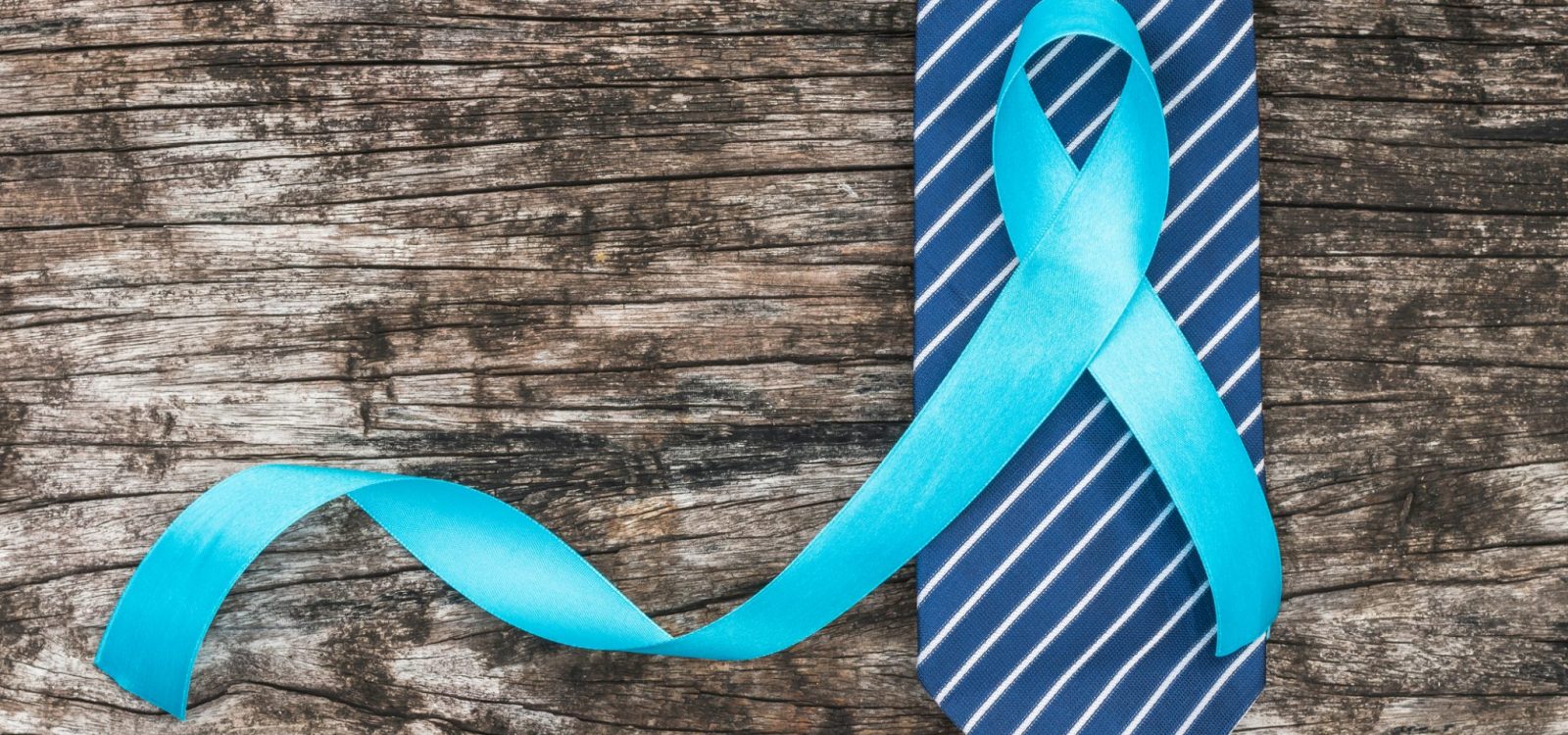
Predicting the short-term mortality of Australian men considering PSA testing
Anthony Lowe outlines the development of a new model for predicting short-term life expectancy based on age and self-reported health data only for use by Australian men considering Prostate Specific Antigen (PSA) testing for prostate cancer.
In 2016 Cancer Council Australia and Prostate Cancer Foundation of Australia published national clinical guidelines on Prostate Specific Antigen (PSA) testing[1]. One of the guideline recommendations is that PSA testing is not recommended for men who are unlikely to live another 7 years. As shown in the figure below, the reason is that Randomised Controlled Trial evidence[2] shows that any mortality benefit from early diagnosis of prostate cancer due to PSA testing is only seen 6 to 7 years after commencement of testing. Men who are unlikely to live this long might suffer reduced quality of life from the adverse side effects of treatment for prostate cancer[3], but derive no benefit in terms of reduced mortality.
The likelihood that an Australian of a given age will live for a certain number of years can readily be calculated from the Australian Life Tables published by the Australian Government Actuary[4] as lx+7 / lx. The percentage of men of a given age who will live for another 7 years is shown in the figure below. Reading from this figure, for example, only 60% of 80-year-old men can be expected to live another 7 years.
The Australian Life Tables 2010-12 are based on Census data and therefore represent the mortality of men and women in average health for their age. Many older men and women have a number of co-morbidities, which can have a significant impact on life expectancy. Hence, to enable accurate advice to be given to men about whether they are likely to live long enough to benefit from PSA testing, the mortality data needs to be stratified by health status and incorporated into a readily available life expectancy estimation tool.
While several relevant mortality prediction tools are available for clinical practice, they have been developed mainly for assessment in the elderly, make only short-term (6-month) predictions, or have been developed for high risk populations. Additionally, a tool based on self-reported health variables that can be easily obtained without physical examination would be easy to use in clinical practice and therefore most likely to gain widespread acceptance and use.
Therefore, we (epidemiologists Grace Joshy, Mark Clements, Emily Banks and Bruce Armstrong, actuaries Anthony Lowe and Leonie Tickle and biostatistician Rory Wolfe) set out to develop a new life expectancy prediction model which could be incorporated into an online tool using age and self-reported health status as inputs. The results of our study, and the three prediction models we developed using data from the Sax Institute’s 45 and Up Study[5], are described in a recently published paper in BMJ Open[6].
Individuals joined the 45 and Up Study by completing a baseline postal questionnaire which included self-reported information and behavioural risk factors, medical and surgical history, functional capacity, self-rated health, quality of life and psychological distress. Data from study participants has been linked to deaths by the Centre for Health Record Linkage.
To develop our model, we analysed 40 variables from the baseline questionnaire as potential mortality predictors using a Cox proportional hazard model[7] with age as timescale. To assess the age adjusted association with mortality for each of the potential predictors we performed univariate analysis using time in study as the timescale. Discrimination was assessed on the basis of ten-fold cross-validated Harrell’s C-index[8].
We found that self-reported health was the strongest predictor of all-cause mortality among men (C-index 0.827). Other strong predictors included physical functioning limitations, quality of life, needing help with daily tasks, previous diagnosis of cancer, smoking, feeling tired, feeling everything is an effort, diabetes, heart disease, BMI, sufficient physical activity, stroke, treatment for osteoporosis, blood clot and Parkinson’s disease.
The coefficient estimates from the three models were used to create risk prediction scores. After stepwise regression, Model 2 included 7 variables and 5 interaction terms with age and Model 3 included 19 variables and 8 interaction terms with age. Internal validation of the prediction scores indicates that each model has good discrimination ability. The C-indices for the three models are 0.828, 0.838 and 0.848 respectively, compared with 0.803 for a model based on age only.
The main difference in the outputs of the three models is the range in short-term survival. For example, the range in the percentage of men remaining alive after 7 years is broader under Model 3 than Model 1. This is not unexpected given the simplicity of Model 1 compared with Model 3. The variation in age-specific 7-year survival under Model 3 is shown in the violin plot below. Both the risk of death and the spread of the distribution increase with age, especially above age 65 years.
The models we have developed represent a credible and easily useable approach to estimating mortality risk over 7 years for use by Australian men considering PSA testing in the context of the national clinical guidelines. An online tool using Model 3 is currently being developed to give men and their doctors easy access to the mortality risk prediction. The strength of Model 1 using only age and self-reported health begs the question “why use a more complex model?” Whilst we have no empirical data with which to answer, we hypothesise that men’s confidence in a mortality prediction tool is likely to be greater the more of their health experience the inputs cover. Model 1 may be perceived as too simple to provide credible information.
The research project was a novel, but excellent, example of how actuarial techniques can be applied to improve public health outcomes.
This research project was co-funded by Actuaries Institute and Prostate Cancer Foundation of Australia.
References
[1] https://wiki.cancer.org.au/australia/Guidelines:PSA_Testing
[2] Schröder FH, Hugosson J, Roobol MJ, Tammela TL, Ciatto S, Nelen V, et al. Prostate-cancer mortality at 11 years of follow-up. N Engl J Med 2012 Mar 15;366(11):981-90 Abstract available at http://www.ncbi.nlm.nih.gov/pubmed/22417251.
[3] The main potential adverse side effects from treatment from prostate cancer are urinary incontinence, erectile dysfunction and bowel problems
[4] Commonwealth of Australia. Australian Life Tables 2010-2012.; 2014. Available from http://www.aga.gov.au/publications/life_table_2010-12/default.asp.
[5] https://www.saxinstitute.org.au/our-work/45-up-study/
[6] Joshy G, Banks E, Lowe A, et al. Predicting 7-year mortality for use with evidence-based guidelines for Prostate-Specific Antigen (PSA) testing: findings from a large prospective study of 123697 Australian men. BMJ Open 2018;8:e022613. doi:10.1136/bmjopen-2018-022613
[7] https://en.wikipedia.org/wiki/Proportional_hazards_model
[8] https://en.wikipedia.org/wiki/Receiver_operating_characteristic
CPD: Actuaries Institute Members can claim two CPD points for every hour of reading articles on Actuaries Digital.