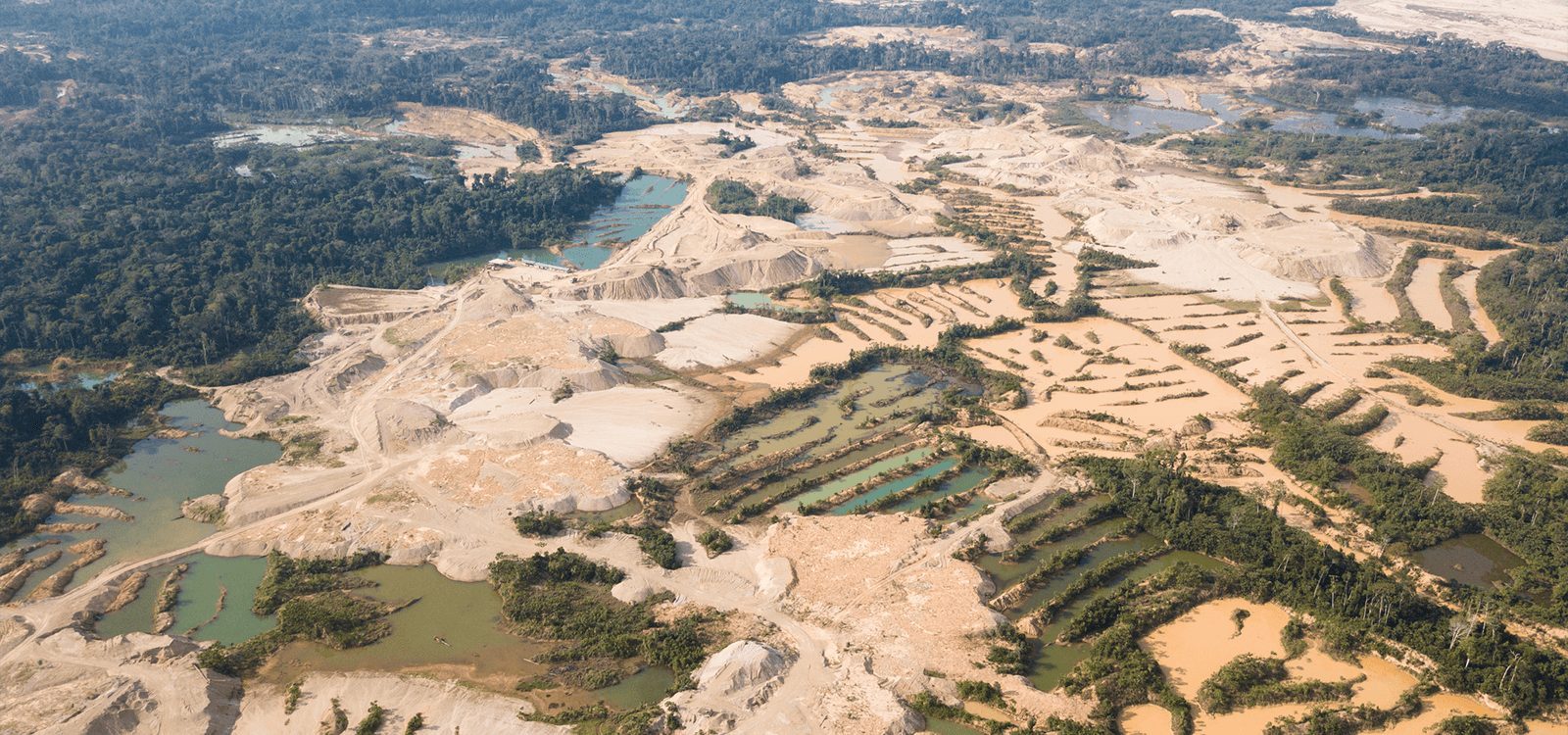
Sustainability and development: How machine learning can counter illegal mining
Illegal mining is an increasingly important issue that sits at the intersection of environmentalism and economic development. It is a powerful symbol for the challenge many developing nations face in taking action on climate change while simultaneously grappling with complex social issues. And perhaps somewhat surprisingly, the actuarial skillset could have a role to play in meeting this challenge.
We used to make our way up from Johannesburg. It would take about five hours by car before we reached Kruger Park, one of Africa’s largest wildlife reserves. Over the course of the drive, we’d see the landscape shift dramatically. First, we’d see the inner city full of tall buildings and coffee shops. Then we’d reach the city outskirts, dotted with shanty towns, persisting from the segregated townships of Apartheid. Finally, we’d reach rural South Africa which largely consists of farmlands and not much else. However, occasionally the grasslands were punctuated by flourishes of industrialisation. We’d see towering steel structures, busy trucks, bulldozers, and cranes – South African mines at work. These sites offer a stark contrast to Kruger Park, which, in my opinion, represents genuine coexistence between humans and nature. Kruger really is an oasis. If you look at a map of mining-licensed sites in South Africa, you can easily identify Kruger as one of the few areas in South Africa with no approved mining zones.
Legal mining remains an active topic of debate in South Africa. While it can be devastating to the environment, it is vital to the economy. However, in this article, I’m going to focus instead on illegal mining, which is unambiguously detrimental. Illegal miners in South Africa are called zama-zamas, a Zulu expression meaning ‘those taking a chance’. Sadly, the saying is justified; it is spectacularly dangerous, with hundreds of deaths over the past few years. Fatalities are only increasing as South Africa’s economy falters and people get more and more desperate. Illegal mining can also cause irreparable ecological damage through toxic contamination, pollution, and the destruction of biodiversity.
Illegal mining is a symbol of the intersecting challenges developing nations face in acting on climate change and promoting economic & social development. Despite their extreme social and environmental costs, they can represent a key income for some communities. This drives a self-reinforcing cycle of poverty and environmental degradation. A cycle which is all too familiar to governments of developing countries.
While it is widely accepted that climate action can generate long term value and economic growth, developing countries often struggle with raising the capital needed to invest in a sustainable future. As a result of this capital constraint, a trade-off emerges in the short-term – between investing in economic development initiatives and taking action on sustainability issues. There seem to be three ways to try to overcome this trade-off:
- Find initiatives that promote both development and sustainability.
- Improve the supply of capital; increase its availability and reduce its cost.
- Improve the efficiency of sustainability initiatives so that more impact can be achieved with less capital.
This article speaks to the third approach, with a focus on illegal mining.
Preventing and closing illegal mines is costly; it involves monitoring vast swathes of land and responding to illegal mining activity when identified. Improving the efficiency of this work – the third item above – could offer significant environmental benefits.
Below, I offer a story of how machine learning can play an important role in delivering efficiency gains that enable governments to get more bang for their buck.
Machine learning and illegal mining
If machine learning can be used to improve the efficiency of illegal mining countermeasures, then it will provide benefits in two ways:
- Improvement in response times. The government can use machine learning models to monitor for operational illegal mines in real-time, drastically improving the pace of their response for a relatively small price. Mining can be shut down faster, limiting damage.
- Discouragement effects. Illegal mining is costly, one needs to get machinery to a remote part of the planet, clear out an area, and hope that there are sufficient rewards to justify the means. If governments made clear that they would stop these mines, i.e. if they published and publicised that they were implementing faster, more sophisticated countermeasures, the value proposition for miners would diminish. This could proactively save some of the vast amounts of money and resources it takes to shut down illegal mines.
Machine learning applied to satellite imagery offers a path to quickly detecting illegal mining activity. This computer vision technology can yield three key benefits:
- Identification of operational illegal mines, the scale of those mines, their locations, and the speed with which they are expanding.
- Ability to perform retrospective analysis to evaluate the success, or lack thereof, of environmental protection laws.
- Ability to monitor the success of rewilding and clean-up of previously closed mining sites and ensure mines are not resurrected.
Now let’s discuss the solution itself. There are three main challenges to building an effective model for this problem:
- Firstly, and most importantly, illegal mines are never officially registered, by design, they are inherently and purposely hidden. This means that there is very little training data with which to build a sophisticated model.
- Secondly, protected regions are massive. In total, they cover around 15.4% of the world’s land mass, more than 30 million square kilometres. That’s about three times the size of Europe. The sheer size of this area poses a computational challenge for advanced modelling approaches.
- Finally, cloud coverage can obscure areas temporarily. So any algorithm needs allowance for this to avoid false alarms.
Methodology
To demonstrate the implementation of an approach, we:
- define in-scope protected areas in consultation with relevant stakeholders;
- procure satellite imagery data for the affected region; and
- divide the in-scope area into zones, each approximately 750 hectares in size.
An example zone, before and after additional deterioration, is shown below.
For each of these zones, we apply two algorithms (with the help of Python’s excellent OpenCV package). The first is a colour segmentation algorithm. To start, a range of tones associated with an illegal mine is specified, this will depend on the terrain that is being searched. In this case, it would range from a light tan to dark brown. The algorithm then segments the image according to whether a pixel falls into this range or not. It darkens and desaturates areas that are not relevant.
This output then becomes the input to the second algorithm, a contouring process. This model looks for delineations or boundaries between light and dark and accordingly draws a distinction between them, in the form of the red lines that you can see in the image below.
Lastly, the area of each region enclosed by these contours is calculated. This process is undertaken for each zone, and a list of suspect zones is then compiled. This list can then be forwarded to an expert to advise on whether the zone does indeed reflect illegal mining.
In the case above, we can estimate the deterioration visible in the ‘after’ image by converting pixels to hectares. The damaged canopy represents an area of around 230 hectares, which is almost the size of Sydney’s CBD.
There are limitations to this type of approach. For example, in estimating the size of the environmental impact, we only see damage to the forest canopy – it is likely that chemicals and machinery from the mining have polluted much more than the area visible from above. Therefore, the 230 hectares in this example is likely a conservative figure.
Conclusion
It is clear that technology will play a significant role in addressing climate change. In particular, machine learning approaches could yield significant efficiency gains. This is especially important for developing countries that often face tight capital constraints.
One exciting aspect of the technology is that it is not just governments that can participate. Conservation and environmental protection groups could also use models like the one described above, with appropriate access to satellite imagery. This represents a data-backed way to hold governments accountable for ecological protection and to apply pressure to an issue that is sometimes swept under the rug.
CPD: Actuaries Institute Members can claim two CPD points for every hour of reading articles on Actuaries Digital.