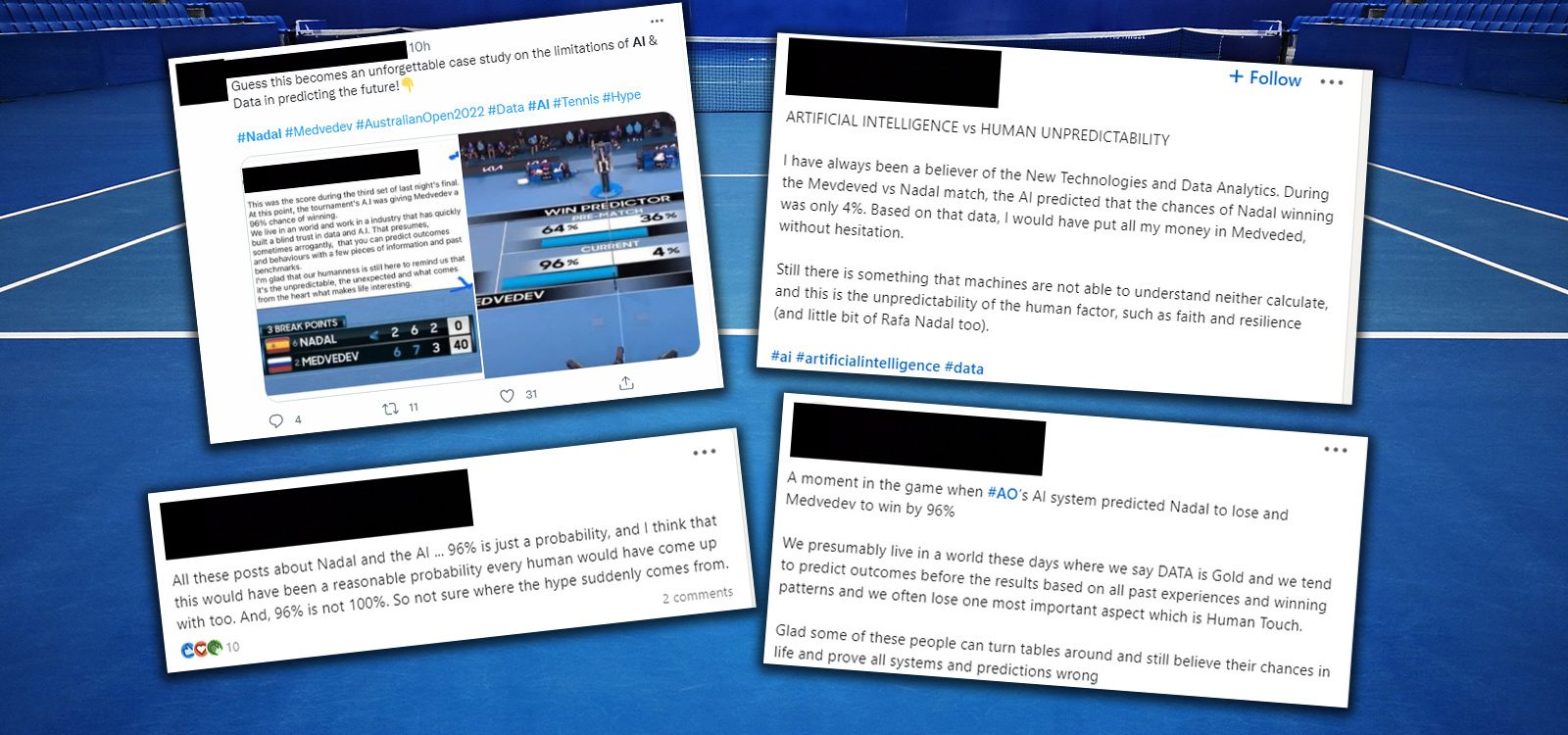
The reaction to Nadal’s “impossible” victory, cognitive biases and data literacy
Chris Dolman reflects on the reaction across social media to an unlikely but plausible event.
I didn’t watch the men’s Australian Open final between Rafael Nadal and Daniil Medvedev on Sunday night, but I’ve been fascinated by a common reaction to it. I think this serves as a useful reminder for data practitioners, actuaries, data scientists and others – most people do not interpret statistics in the same way that we do!
Again and again on social media this week I’ve seen references to a critical point in the game, with Nadal down 0-2, and defending three break points. At this point, an estimate of his chances of victory was listed as 4%, which people claimed was ‘AI generated’ (though I cannot confirm this, that’s the common story).
With the benefit of hindsight, we now know what happened; Nadal came back and won the game, leading many people to declare that this prediction was ‘wrong’ and that he had somehow ‘beaten the AI’. There were some entertaining reflections on the human condition built into some of this.
To show I’m not making this up, below I’ve included a small selection of social media posts that I’ve seen. There are many, many more.
![]() |
![]() |
![]() |
What these posts illustrate is a common form of cognitive bias at play. People will tend to act as though a low probability is zero, then after the fact will blame the ‘incorrect prediction’ when the rare event happens to occur. This combination of probability neglect[1] with hindsight bias[2] is evident in the examples above and many others I’ve seen, with common talk of ‘beating AI’ and the ‘limitations… in predicting the future’..
As we all know, the future is usually uncertain. We need to remember this when interpreting probabilistic outputs of models, which by their nature reflect this uncertainty. In this case, Nadal was given a 4% chance of a win, which in the end was the outcome that emerged. It would be reasonable to talk of ‘beating the odds’, but to suggest this prediction was somehow incorrect is a step too far. One in 25 shots should happen from time to time!
An interesting aspect of this was the suggestion that this occurred because the AI – as a mere piece of software – lacked any appreciation of qualities like ‘human fight and spirit’. Of course, any reasonable person (presumably understanding of such things) if asked to give a prediction of Nadal’s chance of victory at that point would also have given a fairly low but non-zero number.
He was far behind, but there was (as we saw) a small possibility of a turnaround.
4% might well have been a perfectly well-calibrated estimate of his chances at that point – whilst low for a two-horse race, it certainly doesn’t seem too silly to me given the game situation. Some comments I have seen sum this up nicely.
![]() |
So, what is the issue here? Why all the excitement? Why am I writing this? Unfortunately, it’s something that most of us will have seen at some point in our careers, and I think it’s a useful reminder to us of its general application outside of our workplaces, as the world becomes more ‘data driven’. I’ve certainly been in meetings where I’ve had to remind clients that the small downside does in fact exist, might actually emerge and that they should act accordingly. If you assume it won’t happen, you’ll be right most of the time, until the rare tail event wipes you out.
I’ve noticed for some time that many people struggle to think this way and need regular coaching and reminding. But data, stats and even AI predictions aren’t always used today in such a controlled environment – with explanations, caveats and careful discussion – instead, we are putting them on display in the middle of sports games. It should be unsurprising that people misinterpret the information.
So, what can we take from this?
- Levels of data literacy in the general population are lower than you might expect and are further hindered by cognitive biases. If you give people data, estimates or statistical results without guidance, they might not use them as you’d expect.
- People will make closed decisions based on statistical estimates, disregarding uncertainty, then blame the model when things are ‘wrong’. Your models should include guidance to the users against this problem, so at the very least you can fall back on: “but I told you there was uncertainty”.
- You should actively check that model users are interpreting things as you’d expect, even if you’ve given them instructions. Check their rationality.
- If a rare event occurs that you predicted was rare but not impossible, assume people will blame you for being ‘wrong’, and act accordingly!
References: |
CPD: Actuaries Institute Members can claim two CPD points for every hour of reading articles on Actuaries Digital.