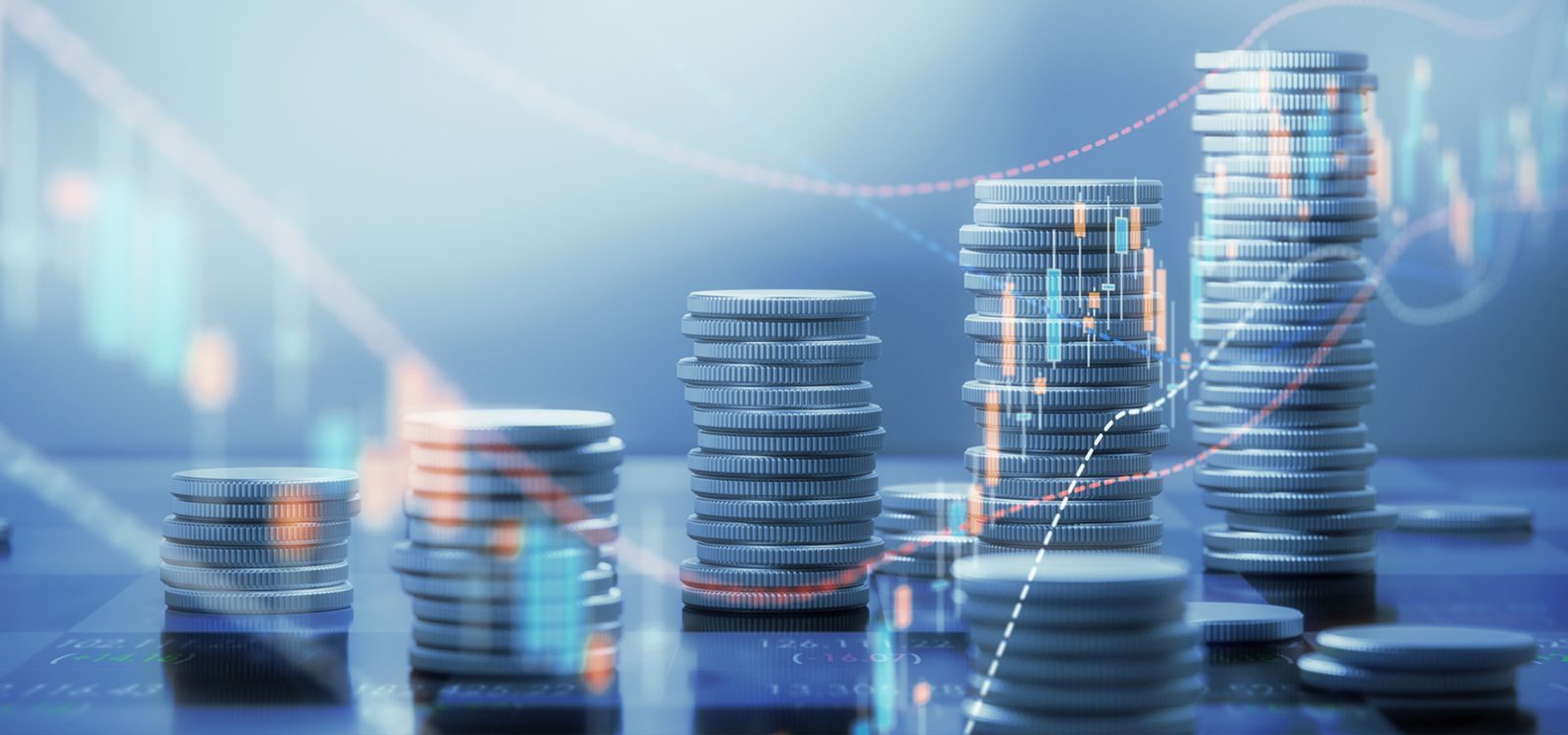
Data – The oil in industry consolidation?
The increase in industry consolidation has heightened the need for clearly defined data models, supporting integration and scalability.
The superannuation industry is experiencing significant consolidation through merger and acquisition (M&A) activity. Various larger funds are acquiring smaller funds and effecting the integration by simply absorbing them into the larger fund’s technology and infrastructure. However, recent market activity has seen the consolidation of some of the larger funds across the country, creating ‘mega’ funds[1] like those prevalent in Europe and Canada. For these mega funds, data integration can often be one of the biggest challenges. Transferring the assets and change of control activities are often the primary focus of a Successor Fund Transfer (SFT). However, APRA also clearly expects an RSE licensee to have a robust data risk management process during an SFT[2]. Is there a (relatively) easy way to future proof an organisation for this?
Data challenges and opportunities
Front office teams are concerned about having ‘agile, dynamic and real time’ data[3], especially the larger funds, where trading desks are being insourced. Having insourced trading desks provided a competitive advantage during the 2020 COVID-19 turmoil compared to peers who rely on outsourced capabilities for critical functions such as liquidity management[4]. The speed at which these funds were able to respond proved to be a real differentiator in managing member risks and take advantage of opportunities as they arise. Unless a fund has a clearly defined data model ahead of integration through M&A activity it runs the risk of disrupting these competitive advantages it might have and potentially not realise the benefits of scaling these capabilities in a timely manner.
Middle and back off teams are tasked with supporting the governance functions of the funds, amongst other activities, through clearly assessing risk exposures and reporting on performance to support fiduciary oversight. These teams tend to be dual hatted as they also provide valuable management information to inform daily decision-making processes. Not having a clearly defined vendor and custodian agnostic data model ahead of any integration provides the challenge that reporting deliverables are delayed or even interrupted if time series data is not easily mapped for changes associated with these integration activities such as custodian transitions for example.
Data as an asset
Market consolidation has an interesting dynamic with the management and use of data. The rise in machine learning and AI has seen a surge in demand for skillsets such as data scientists and actuaries for more than a decade. However, the analysis and insights were often limited to those with the sought-after skillsets, which was further exacerbated by the fact that most of the data used were often locally managed and not readily available to the rest of the organisation. More recently organisations have started to recognise their data as an asset, often using the analogy that it is the oil which keeps the wheels turning. Larger organisations have the potential to leverage that larger dataset across its operations.
As a result, most organisations have embarked on some form of data strategy with various levels of sophistication and progress on their journey towards harnessing their data as an asset. The strategies often consist of one or more of the following:
- Data Governance;
- Data Architecture; and
- Data Delivery.
Whilst data governance supports the embedding of clearly defined data practices and the identification of roles and responsibilities, such as data stewards and data custodians, it is data architecture that either supports or hinders data integration. Data architecture has many facets, including how data is to be integrated, stored, transformed and used in systems as well as throughout an organisation. Underpinning strong data architecture is a clearly defined data model which considers the different data assets, their relationships, attributes and lineage.
In the case of a superannuation fund, having a clearly defined data model (that is vendor and custodian agnostic) supports the integration with different providers to enable a best of breed approach when considering M&A activity and avoids disrupting business activities through rushing custodian transitions etc.
A clearly defined data model is the foundation for ultimately delivering data to consumers and end users. In the absence of having an organisation specific data model, quite often data definitions from vendors and custodians are adopted, which makes it extremely challenging to upgrade, migrate, transition, or transform data over time.
Data demands
Irrespective of the form an SFT takes on day one, there continues to be an ever-increasing demand for data from funds. The first and most important requirement is the internal demand for data to manage and inform the day-to-day activities of the fund. The days where data could be completely outsourced and periodically received and relied on are numbered as the onslaught of COVID-19 highlighted the need to have access to readily available data to deal with the black swan events and act in members’ best interest.
Following this, the regulatory submissions continue to increase, with more disclosures[5] set to come. Members also demand greater transparency and insights into fund activities, especially in areas such as socially responsible investing which has sparked significant activism. Not to mention the continued focus on peer relative performance and the associated data demands from rating agencies to differentiate and assess fund performance.
The uptake in unlisted assets has also provided its own data challenges, as quite often these assets are not custodially held.
The increase in demand continues to reduce the time available to source, transform and analyse the data. Having a clearly defined data model supports the creation, sourcing, integrity and quality of the data being provided, reducing the need for manual intervention and excessive duplication for sourcing data to validate and check it.
Seeing the big picture
With industry consolidation increasing, the complexity of the SFTs continues to rise (at least whilst the integration implementation is executed). Clearly defined data models provide an end-to-end view for solving such business challenges and provides a roadmap for integration.
For many organisations data has become an integral part of their end-to-end decision-making process, embracing the development of clearly defined data models. These organisations are able to quickly navigate the changing landscape. However, for some organisations data remains a ‘first cog in the process’ mentality, with little to no investment made in maturing their data capabilities or considering data as a ‘back-office’ problem. These organisations also often consider data as a commodity that can be outsourced and treat it as a cost saving activity. Such organisations run the risk of quickly becoming obsolete, with little to no probability of scaling their solutions.
CPD: Actuaries Institute Members can claim two CPD points for every hour of reading articles on Actuaries Digital.