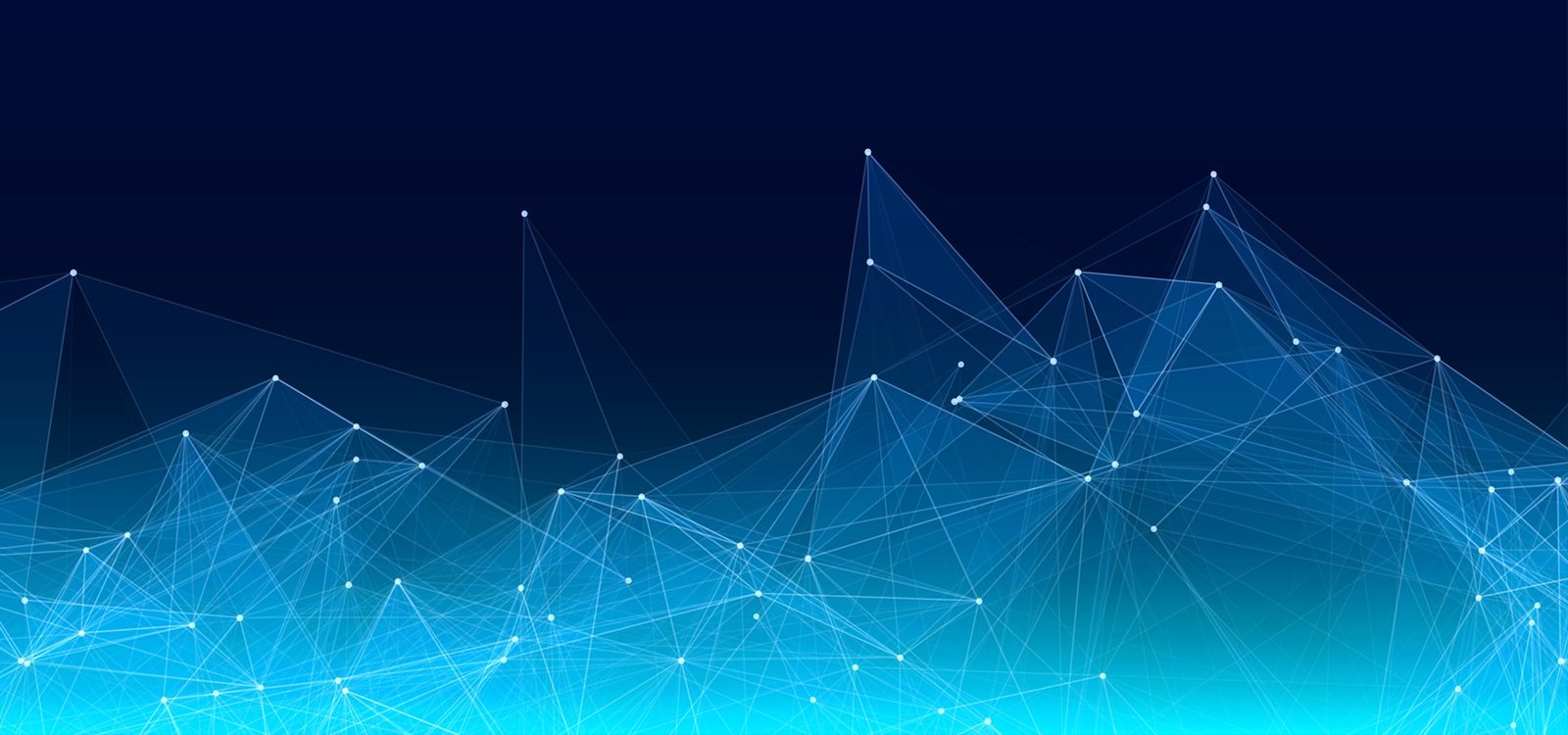
Let’s stop talking about “risks of AI bias”, and instead start deciding what we want the world to look like
I have been part of several panel discussions on artificial intelligence (AI), data and tech ethics in recent months. Each time, usually about halfway through, the following sort of question is posed: “What do you think we should do to manage the risks of AI bias?”
This short essay is a more considered answer than my usual ‘top of mind’ response to this question. I have struggled to solidify my thinking on this question for a while, and I think this is because the question itself represents part of a bigger issue. There’s a mistake of logic within it, and its ubiquity as a question highlights how common this mistake is. We need to stop asking this question and start asking better ones. Or, better still, start a proactive discussion about the world we want to live in and the norms we wish to encode to create that world.
Why the question is the wrong one
First, there is a big problem of language within the question. Let’s stop avoiding the actual topic and call a spade a spade. ‘Bias’ has become a codeword for discrimination – this needs to stop. It does nobody any favours to avoid a direct discussion of difficult topics by way of codewords and doublespeak. In saying this I fully acknowledge that ‘bias’ can mean far more than just discrimination, but that broader definition is never what this question is getting at.
Second, we really, really need to stop calling this a ‘risk’. The word ‘risk’ suggests some amount of chance or uncertainty, some unlikely but plausible negative outcome which we need to take steps to detect and manage. This is utterly wrong. There is no uncertainty of this sort here – some form of indirect discrimination will almost certainly exist, in almost all decision contexts.
The question is only by how much, in what form, and against whom. It is something the Actuaries Institute has already said, quite plainly, to the Australian Human Rights Commission in a recent consultation[1]. It is vanishingly unlikely that a decision mechanism is completely uncorrelated to any of the many, many protected attributes covered by discrimination law, most of which are never seen by a decision process. We must assume that all decisions are indirectly discriminatory unless proven otherwise, rather than treat this as a potential outcome to be detected and then – only if detected – managed.
A better question, and a way forward
So how can we improve our dialogue on this important topic?
First, we need to openly acknowledge that the existence of some (indirect) discrimination need not always be a problem. If discrimination is everywhere, surely sometimes it must be legitimate? The law acknowledges this: indirect discrimination is allowable in many countries if a ‘reasonableness’ test (variously defined) for the indirectly discriminatory procedure is passed. The Anti-Discrimination Working Group’s paper from last year’s Actuaries Summit outlines how this works for Australia in some detail.[2] Your AI process (or, indeed, any decision-making system) needs to pass this test, since there will always be some discrimination of some sort occurring.
I think we tend to revert to the initial, flawed, question because in many cases we have no clear conception of what a ‘good’ decision looks like. We have not worked out, precisely, how to divide the space of potential decision procedures into ‘reasonable’ and ‘unreasonable’ ones. The ubiquity of the initial question demonstrates an important problem: we often haven’t even worked out that this is the real issue at hand. Even taking this initial question as posed, we surely cannot hope to ‘manage the risks of AI bias’ without even knowing what would be considered ‘reasonable’, or even starting to think to ask that question.
So, it’s simply not good enough for us to continue framing ‘AI bias’ as a ‘risk’ to somehow be managed. We need to flip this thinking entirely on its head and ask instead: “How ought this form of decision be made?” This always requires a discussion of when, in what form, and how any discrimination should occur. This is not a justification for, but rather an explicit promotion of certain forms of discrimination as the socially correct outcome. To build the world we want, we must describe what that world ought to be, warts and all, not manage some perceived risks of our imperfect world measured against an ill-defined ideal.
What needs to happen, then, is an ongoing societal discussion about this second, better, question across material decision categories.
This needs to be a properly organised, multi-stakeholder debate. It needs to be grounded in an acceptance that in most cases we haven’t yet adequately answered the hard questions that, perhaps, we ought to have already answered – and this is everyone’s fault.
What about our familiar area of insurance pricing? Pleasingly, we can see some semblance of this societal discussion already. We have been having difficult conversations about ‘fair pricing’ for centuries, and plausibly this is because it has long been data-driven, necessitating some precision. We have debated whether risk pricing is appropriate, and the affordability issues this can create for some. We have debated the use of genetic information for life and health. We have changed our mind on gender as a rating factor, at least in the EU. Other examples abound. It’s been a constant discussion and might never end – but at least it is occurring.
Recognising that this is ongoing, without a complete answer, could anyone truly say today that they know, with absolute certainty, what the correct definition of a ‘good’ insurance pricing system is? Could they confidently divide all potential pricing systems into ‘reasonable’ and ‘unreasonable’ ones? Perhaps not, but I do think we can be at least somewhat confident in the answer for at least some situations, and we can take comfort that such discussions will continue. For example, motor insurance prices are generally based on vehicle type, since Ferraris cost more to repair than Fords. This is intuitively thought of as ‘reasonable’ information to rely on – few would even think to question it. However, this certainly creates some indirect discrimination against someone, unless we believe, implausibly, that all types of car are owned by a representative sample of the population. But we don’t ask whether we are ‘managing the risk of bias’! It’s not a risk to be identified and managed, it’s something that is there and accepted – because society has determined that it’s a reasonable thing in this context.
In many high-stakes contexts where data-driven processes are relatively new, this sort of discussion has barely begun. But rather than have it, we are going around asking each other about ‘managing the risks of bias’. It’s the wrong question. Bias – or discrimination – will always be there whenever a decision is made. But how ought that decision be made?
This highlights the potential value of the burgeoning AI ethics field – it can force some precision on difficult but essential normative questions we have historically glossed over, particularly in traditionally human decision processes. However, this value will only be realised if we can begin to ask better questions. Not reactive questions couched in the language of risk management, but proactive questions about our desired norms and values.
Let’s get on and do it.
References |
The views and opinions expressed in this article are those of the author, and do not necessarily reflect those of the Actuaries Institute, the author’s employer or any other associated bodies.
CPD: Actuaries Institute Members can claim two CPD points for every hour of reading articles on Actuaries Digital.