How to proxy the unmodellable
At the 2018 GIS, a paper prepared by Alan Xian was awarded the Taylor Fry Silver Prize. In this article, Alan outlines key highlights of their paper on Analysing granular insurance claims in the presence of unobservable or complex drivers.
This article is based on our 2018 GIS paper How to proxy the unmodellable: Analysing granular insurance claims in the presence of unobservable or complex drivers. A related paper considering count models in more general contexts can be found here.
I think modelling is a very difficult thing. It’s glamorous and fun, but underneath it all, you know your flaws, and those are what you focus on.
– Pattie Boyd
Analysing data is hard work. Practitioners are spoiled for choice by an ever-expanding toolkit for cutting up and restitching their datasets into sharp, chic showcases. Perhaps sometimes this makes modelling more difficult due to analysis paralysis over the best way to approach a problem. As savvy actuaries (or actuaries-in-training), let’s not get lost in the detail and take a momentary step back.
Why do we model? Undeniably, the outputs are always part of the reason. However, the journey to understand relationships within the data is often equally important. Connections between inputs and outputs, as well as the inputs themselves, help modellers explain the end results. All models might be wrong, but the process is generally valuable.
So then what are we modelling? The data landscape that actuaries navigate has been rapidly evolving, with datasets growing in multiple dimensions. The number of records is increasing, along with the number of covariates associated with each record. Furthermore, the granularity of data records available to us has also increased. For example, near-exact motor collision times (amongst many other things) can be obtained (see FARS in the US and RSD in the UK).
This makes analysis a bit tougher. The three-dimensional box of data (length, breadth and granularity) is now larger, allowing new trends and interactions to emerge. We want the best tool for the job of extracting this information, but “best” is not solely based on accuracy and precision. Consider the diagram below. With great complexity comes great potential for model and parameter error. In most actuarial situations, interpretability is also front-of-mind. We also must be able to obtain the data required and have the technical capability to implement the model. Finally, applications need to attain a certain level of computational efficiency because a four-month calibration process to estimate quarterly reserves is an unproductive exercise. Ultimately, the idea of materiality underlies all practical considerations. Is the result worth the effort required? Our task is to find the optimal model to manage these trade-offs, involving not only comparisons between different model types but also parameter and covariate selection.
The MMNPP Model Framework
In our paper, which was awarded the Taylor Fry Silver Prize at GIS 2018, we outline a procedure to assist in this endeavour by examining not only what is directly analysed by the practitioner’s chosen model, but also proxying drivers that are left unmodelled. This could be due to their inherent unobservability or the fact that these drivers are too complex.
We provide an example in Section 4.1 of the paper. A relationship between rainfall and motor frequencies is suggested by the statistically significant correlation value. However, proper modelling could be prohibitively difficult. It might be necessary to account for a myriad of other factors such as population densities, road conditions, and other demographic/geographic/meteorological considerations.
What is one to do here? Instead of either expending excessive effort or ignoring it completely, we propose the following two step procedure. Firstly, a model is selected to incorporate whatever data features can be feasibly included. A scaling procedure then isolates and removes the effect of these modelled elements. The residual information is then grouped into regimes using a Markov chain, which proxies the aggregated impact of all unmodelled factors.
This framework is based on the Markov-modulated non-homogeneous Poisson process (MMNPP) which splits into the two components outlined previously:
- The frequency perturbation measure, which is what the practitioner has chosen to explicitly model. This is flexible, allowing the use of any model type.
- The hidden Markov chain, which serves as a proxy for the “residual” information that was left unmodelled due to materiality, complexity, interpretability or implementation issues. (Note that, heuristically speaking, this chain models the expected “residual” rate of claims and not the claim arrivals themselves).
Various theoretical and computational innovations were developed for efficient calibration on standard computing software. Details for interested (or insomniac) readers can be found in the appendix of the paper.
A Toy Implementation Example
We use a toy example to demonstrate implementation and provide some intuition. Consider the following figure with a blue line depicting daily claim numbers. The red line plots the perturbation measure. This is a relativity of the chosen model’s frequency prediction each day relative to a designated base day (Day 1 in this case).
The raw claim numbers are then scaled by the perturbation measure to obtain “residual” claim numbers. These numbers contain leftover information after having accounted for all explicitly modelled factors.
A Markov chain is then fitted to identify regimes. The number of regimes can be chosen subjectively or utilising the statistical procedure detailed in Section 8.5. In this example, we assume there are three regimes (low, medium and high residual frequency) that are toggled between over time.
Note that it can be hard to link the regimes to specific causes, as the Markov chain represents the aggregated effect of all unmodelled drivers of the observed experience. However, we show later that inference is still possible.
Finally, after rescaling the Markov chain frequencies by the perturbation relativities, we can generate closer predictions relative to the non-homogeneous Poisson prediction due to the increased information that is incorporated (see Figure 5 below).
An Australian Motor Case Study
This case study investigates daily motor claim counts based on data from a Linkage project. Full details are presented in Section 8 of the paper. The modelled dataset excludes catastrophe (CAT) claims.
Selection of our model was based on both domain knowledge and statistical analysis. The final chosen model was an over-dispersed Poisson (ODP) GLM with the following covariates (bucketed for parsimony):
- Number of policies in force (as an offset)
- Various forms of periodic variation
- Weekday/Weekend
- Public holiday
- Month
- Day of month
- Days since beginning of investigation period
The Markov chain is fitted using four regimes (1 – Low, 2 – Normal, 3 – High, 4 – CAT-like) with residual frequencies (135, 177, 204, 518). Figure 6 shows a plot of the most likely regimes per day and provides further insights.
Firstly, there is a day of such high residual frequency that it warrants its own regime. This data point could then be further examined.
Secondly, the process generally remains in the normal regime with occasional jumps to the low and high regimes. We could form a hypothesis as to what drivers (no pun intended) underlie these regimes (significant rainfall?) and test it quickly using, for example, correlations with the Australian Actuaries Climate Index.
Lastly, the process jumps down to the low regime somewhat periodically. Upon further investigation, these dates correspond to the 27th to the 31st of December each year. Can you guess why?
Investigated effects can be incorporated into the exposure component if deemed useful and then the whole process can be iterated in a feedback loop until the modeller is satisfied (or has run out of time!). All remaining unmodelled effects are then captured in the hidden Markov layer of the framework.
Conclusion
In any real-world scenario, there is always more in the data than we can explore. The important question is whether this matters. Sometimes, the existing modelling assumptions applied will be adequate. However, it becomes increasingly likely that new effects should be considered as the box of data grows to include “new” intra-data relationships that were previously masked by aggregation and discretisation. Domain knowledge will have to grow concurrently with datasets. So, the next time you take your perils model for a strut down the catwalk (sorry!), it might be worthwhile to quickly check if anything was left out.
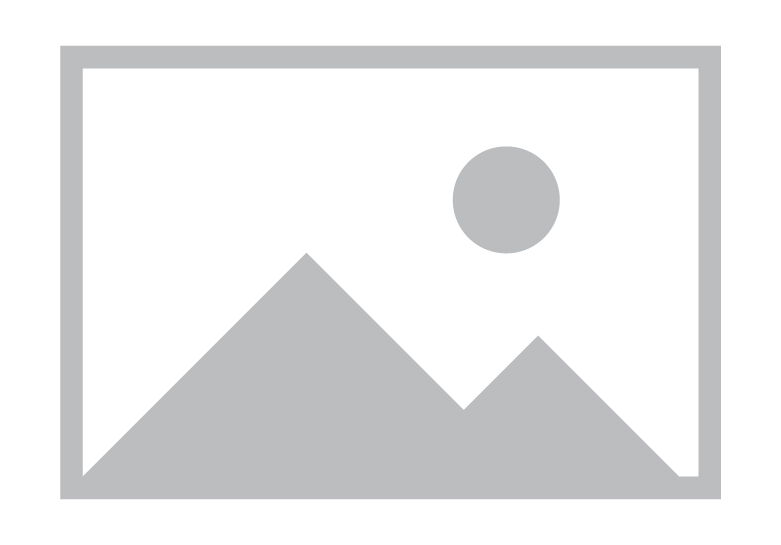
CPD: Actuaries Institute Members can claim two CPD points for every hour of reading articles on Actuaries Digital.