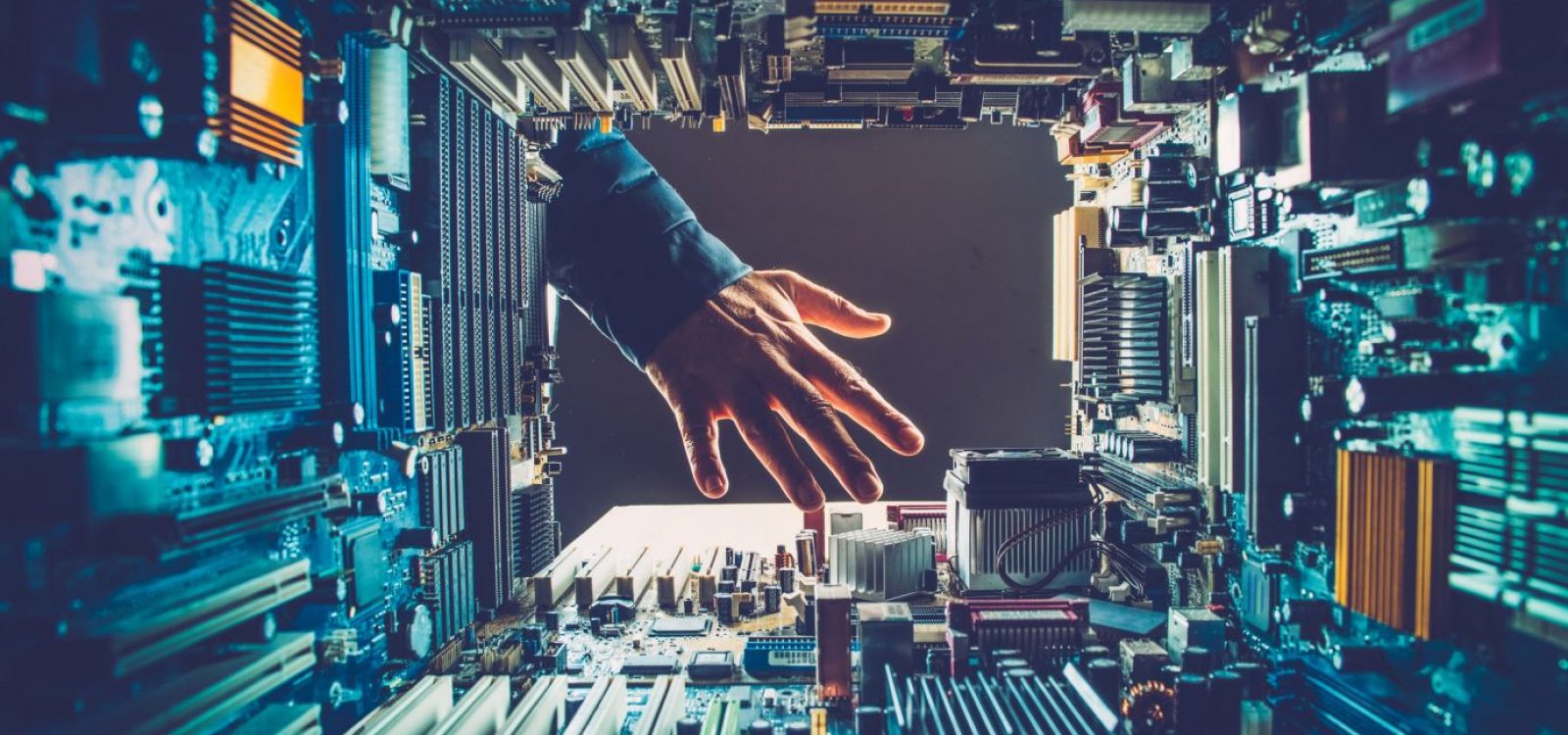
Normal Deviance – In praise of the GLM
In this column, we celebrate the success of the generalised linear model and explore why they are so popular. In its companion column, we’ll look at the model’s limitations and ask whether there are better alternatives.
I consider myself fortunate to have a job where I get to fit lots of models to solve a range of problems. The most class of model I use is the generalised linear model (GLMs), and I think that’s true for lots of people doing actuarial or analytics work. GLMs are a class of regression models that have become the de facto standard across insurance, government and many other industries. The definitive textbook was written in 1989 and the nineties saw widespread adoption that has endured.
Why have they proven so popular? Let’s run through the main reasons:
- Flexible response variable: GLMs can model a wide range of outcomes including binary (did a customer leave?), count (how many crashes this year?) and continuous (what did the claim cost?). Having a common framework for such a range is powerful.
- Multivariate accuracy: While taken for granted today, combining multiple effects in a way that produces consistent and accurate predictions was a big deal. Even today, GLMs give reliably good accuracy, often off the back of relatively few parameters.
- Inference: The ability to formally test hypotheses, including the statistical significance of a predictor variable, is important. It means model can be used to generate insight as well as predict. Often being able to say an effect is not important is just as useful as finding something that is.
- User control: Perhaps more than any other multivariate modelling approach, the user oversees the crafting of effects in a GLM. This allows as us to impose constraints on the model (such as smoothness or monotonicity of an effect). This can be useful when it comes to model updates too—the same structure can be imposed on new data.
- Explanation and interpretation: Because a GLM is typically a smallish set of parameters, effects can be given a clean interpretation as to how it impacts the predictor (‘each year of age adds 2% to claim cost’).
- Extensibility: Linear models and their GLM extensions have become a natural starting point to extend how we model. For example, GAMs are a variant that automate nonlinear predictor effects, while penalised regression approaches such as the lasso have allowed regression models to be applied to datasets with more predictors. Mixed models allow random effects with priors to be incorporated. The GLM framework remains an important starting point for research and development.
Few other predictive algorithms have as much to commend them. In fact, many modern algorithms could be improved by adding in some of the strengths enjoyed by the GLM. Regardless, I think the GLM will remain a reliable workhorse for many years to come.
CPD: Actuaries Institute Members can claim two CPD points for every hour of reading articles on Actuaries Digital.