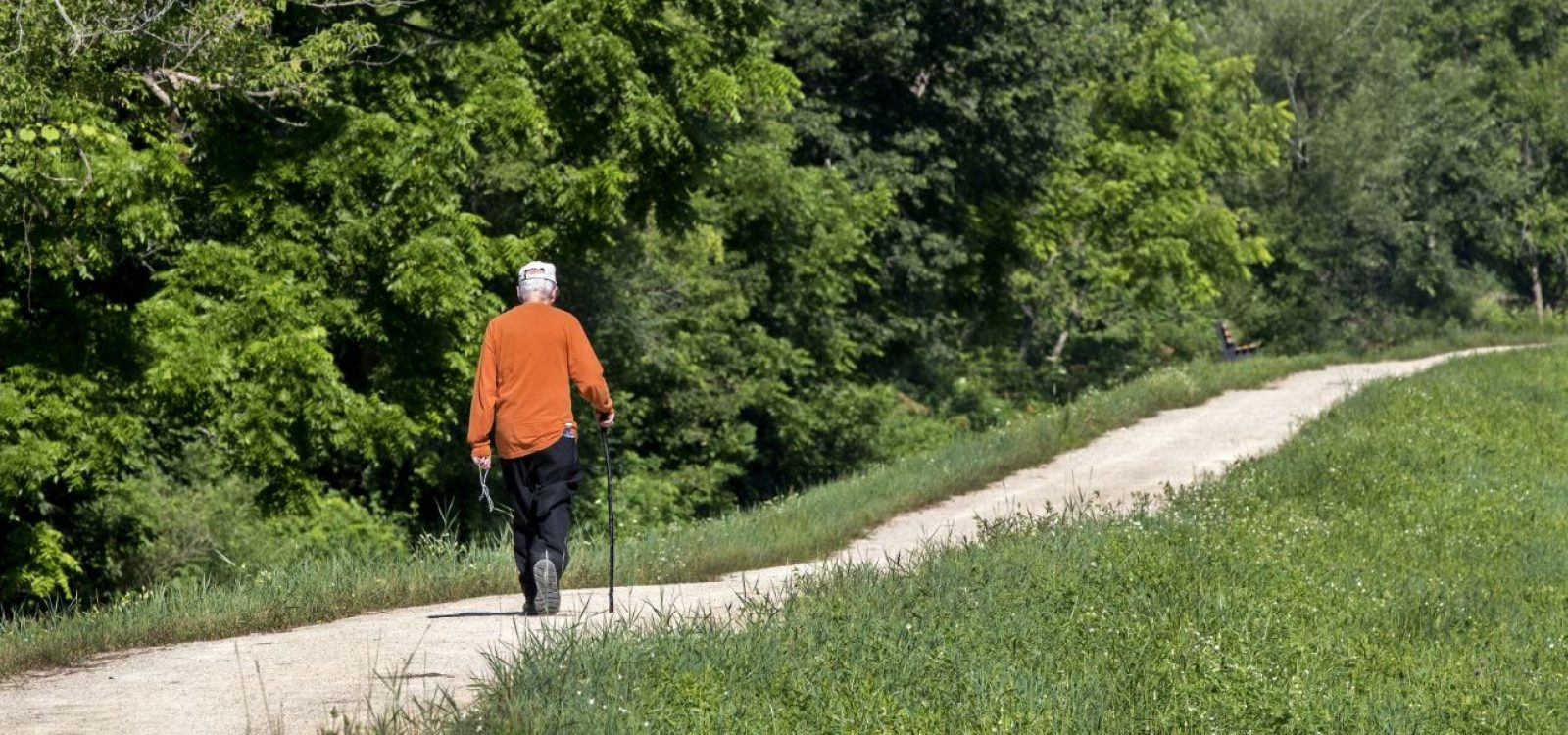
Want to know if you’re likely to be alive beyond 2020? Ask yourself!
Anthony Lowe and Leonie Tickle outline a new online tool for estimating life expectancy based on age and self-reported health status.
Looking around at family and friends as they get older how often have you thought “Fred’s going to live forever” or “I don’t think Bill will be with us much longer”? It turns out that there may be more actuarial science behind those subjective assessments than you realise. This is the story of how we discovered that science.
It is common for clinical practice guidelines on cancer screening to recommend an upper age limit at which testing should cease. The reason for this is that people with a comparatively short life expectancy may not live long enough to gain any benefit from early diagnosis of cancer.
The recently developed Prostate Cancer Foundation of Australia-Cancer Council Australia guideline on the PSA (blood) test for prostate cancer makes the following recommendation:
Since any mortality benefit from early diagnosis of prostate cancer due to PSA testing is not seen within less than six to seven years from testing, PSA testing is not recommended for men who are unlikely to live another seven years.
That advice is all very well in theory, but how will Fred and Bill form an objective view as to whether they are unlikely to live long enough to benefit from PSA testing?
From an actuarial perspective the probability that a man in average health will survive seven years is an easy question to answer. It is readily calculated from the Australian Life tables as lx+7 / lx. However, prostate cancer is an older man’s disease, with an average age at diagnosis of 68, and many older men (and women) have a number of co-morbidities which can have a significant impact on life expectancy. Hence, ideally the mortality data would be stratified by health status to enable more accurate advice to be given to a man about whether he is likely to live long enough to benefit from PSA testing.
We (epidemiologists Grace Joshy, Mark Clements, Emily Banks and Bruce Armstrong, and actuaries Anthony Lowe and Leonie Tickle) set out to tackle this problem using the Sax Institute’s 45 and Up Study, a large-scale cohort study of 267,153 men and women in NSW aged 45 and over. Our aim was to develop a model of expectation of life which could be incorporated into an easily useable online tool for estimating life expectancy based on age and self-reported health status.
Individuals joined the 45 and Up Study by completing a baseline postal questionnaire which included self-reported information and behavioural risk factors, medical and surgical history, functional capacity, self-rated health, quality of life and psychological distress. Data from study participants has been linked to deaths by the Centre for Health Record Linkage.
To develop our model, we analysed 40 variables from the baseline questionnaire as potential mortality predictors using a Cox proportional hazard model with age as timescale. To assess the age adjusted association with mortality for each of the potential predictors we performed univariate analysis using time in study as the timescale. Discrimination was assessed on the basis of ten-fold cross-validated Harrell’s C-index. Based on this analysis, 21 variables including age were selected for the model. We also developed two simpler models using a) 11 variables including age; and b) age and self-reported health status only.
For validation and calibration, we compared hazard predictions from the 45 and Up Study weighted by covariate patterns from the Australian Health Study with the observed mortality rate for Australian males in 2012. Finally, we incorporated longevity improvements using the Booth-Maindonald-Smith variant of the Lee-Carter model fitted to Australian male mortality data for the years 1970 to 2011.
… And after this complex statistical analysis, what did we find?
The strongest single predictor of short-term mortality was the answer to the simple question – “in general terms how would you rate your overall health – excellent, very good, good, fair or poor?” In terms of ability as a predictor this was followed by questions about mobility including limitation in climbing several flights of stairs, walking one kilometre and limitation in moderate activities such as pushing a vacuum cleaner and playing golf. As might be expected, other questions including history of diabetes, heart disease, Parkinson’s disease, stroke and smoking status also feature in the model. All three models gave better prediction of short-term mortality than age alone with the simplest model – age plus self-reported health status – performing well compared to the more complex models.
So, back to Fred and Bill. Why do you think Fred’s going to live forever? Because he tells you he feels in excellent health, he has no difficulty climbing stairs and even though he’s 70 still plays golf twice a week. Bill on the other hand, even though he’s five years younger than Fred, always complains about how poorly he feels, has very poor mobility and smokes like a chimney.
Our online tool, however, goes beyond this simple qualitative assessment of Fred and Bill’s health to quantify the effect each element of their self-reported health status has on their short-term life expectancy. The tool will be published later this year a part of a broader decision aid on PSA testing for men and their doctors.
This paper was in part funded by the Actuaries Institute.
CPD: Actuaries Institute Members can claim two CPD points for every hour of reading articles on Actuaries Digital.