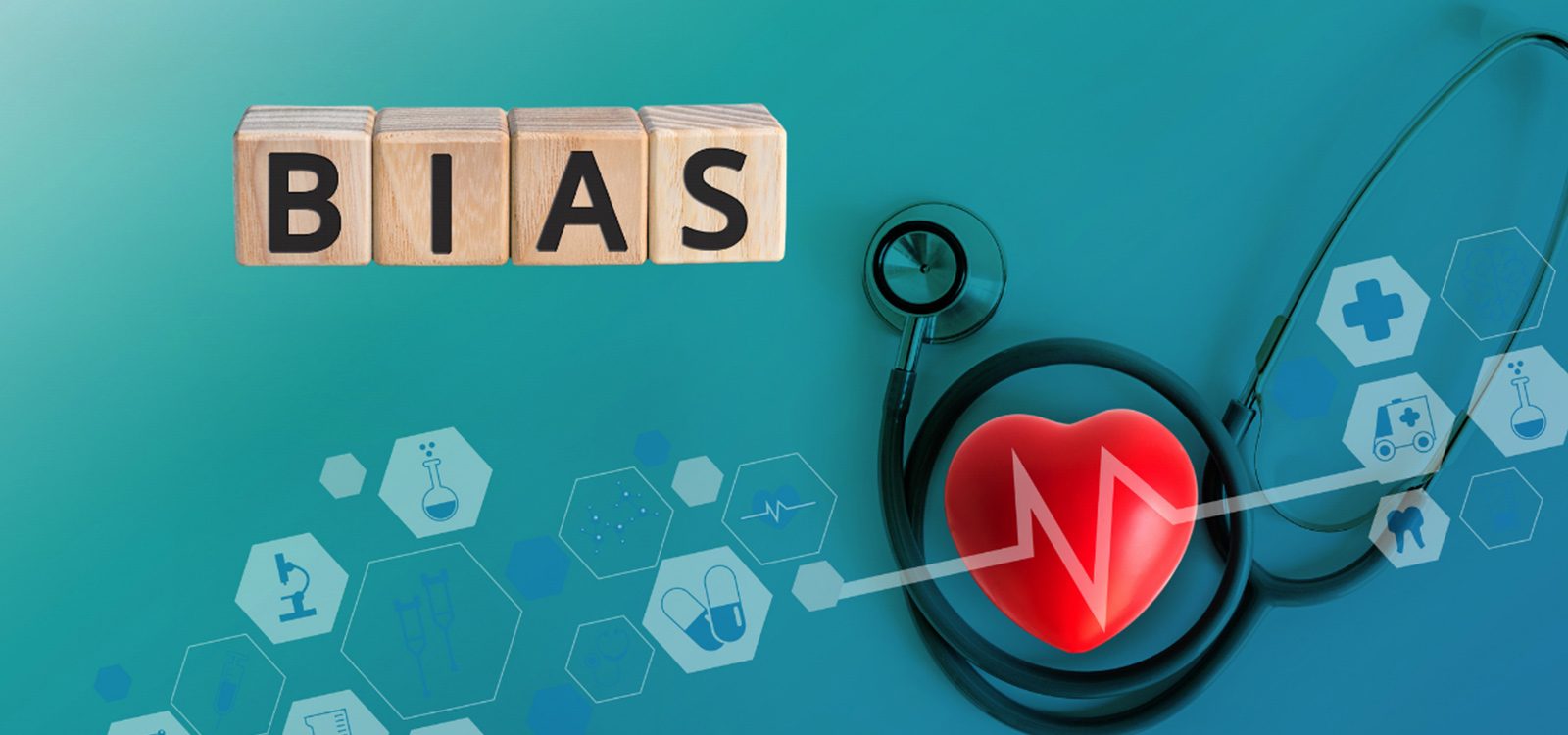
Racist soap dispensers and other tales of health measurement bias
In early-2022, UK Health Secretary, Sajid Javid, put the topics of selection, sample and measurement bias in life and health insurance under the microscope by announcing an investigation into a potential bias in oximeters. Specifically, whether bias was a contributing factor to worse outcomes for ethnic minorities during the COVID-19 pandemic. While, on the face of it, this is an isolated incident, it is actually more commonplace than you may think.
Selection or sample bias occurs when a dataset does not reflect the realities of the environment in which a model will run. Measurement bias occurs when the data collected for training differs from that collected in the real world, or when faulty measurements result in data distortion. These types of bias are discussed in this article.
This topic gained prominence earlier this year when Sajid Javid announced an investigation into a potential bias in oximeters and whether this was a contributing factor to worse outcomes for ethnic minorities during the COVID-19 pandemic. While, on the face of it, this is an isolated incident, it is actually more commonplace than you think. Bias exists in many facets of our life and, in the rush to digitalisation, is often not given enough consideration or adjusted appropriately. This article considers some of the areas where bias overlaps with the provision of life and health insurance.
Biases in medical equipment and treatment
Oximeters, used to measure the amount of oxygen in the blood, determined which COVID-19 patients in UK hospitals were ventilated[1]. NHS England and the Medicines and Healthcare products Regulatory Agency (MHRA) are investigating whether pulse oximeters overestimate the amount of oxygen in the blood.
An oximeter passes light through the skin and, based on the amount of infrared light, determines the level of oxygen in the blood. However, most oximeters on the market today were initially calibrated for white skin, and this inherent bias means they can produce errors more often for non-white people. A study in the USA, of 10,000 patients, suggested pulse oximeters overestimate blood-oxygen saturation more frequently in black people than white people.
A healthy oxygen saturation level is 92-96%. In this study, some patients registering this level via pulse oximetry had a true saturation (recorded by the arterial blood-gas measure) of less than 88%. For black participants, this over-estimation error happened at three times the rate of white participants.
This method of light is also used to operate automatic soap dispensers[2], so there are racially biased soap dispensers out there too!
Wearables are the commercial, non-invasive blood oxygen level measuring product that also provide sleep, exercise and health measurements. Their data is often factored into underwriting decisions or even used as a measurement device in some clinical trials. They can also be used to determine who receives incentives or lower insurance premiums for ‘healthy’ behaviours when rewarding wellness. But is their output reliable?
While Apple watches use infrared and green light to get more accurate measurements of heart rate, many smart watches use solely green light monitoring as it is cheaper to use and less sensitive to motion error than infrared sensors. However, with a shorter wavelength, green light is less able to penetrate melanin and is less accurate taking readings on darker skin tones.
Additionally, the skin tone scales used to measure the efficacy of the wearables is outdated and insufficiently detailed.
The Fitzpatrick Scale, developed in the 1970s, is commonly used3. This scale is a subjective scale with individuals classified into only six different skin tone categories based on perceived skin color, rather than an objective measure of how light actually bounces off of the skin, causing errors in assessing the effectiveness of these devices.
Wearables are uncertified devices, unlike the oximeters used in medical settings and they are often calibrated using the sample population the developers have access to, often the Silicon Valley population which is not a diverse, fully representative training set given the high proportion of white and Asian males.
Some wearables are prone to movement error when measuring (a problem for infra-red methods), a problem not experienced when reading the level using a finger rather than a wrist. Fitbit and Garmin, for example, have disclaimers indicating that they are not for medical use, highlighting the still-evolving nature of wearable data.
A balance needs to be found between cheaper and accessible technology. Perhaps using it to indicate there may be a problem followed by triage/medical assessment by a medical professional trained to accurately interpret the readings using the knowledge that it produces bias for certain members of the population and adjusting appropriately for this.
The rise in the use of telehealth and virtual medical care due to COVID-19 has led to wearable devices aiding individuals managing and reporting their own health status. Historical biases and assumptions underlying algorithms used in machine learning and artificial intelligence can be locked in with the biases inherent in the product itself, which leads to individuals struggling to access proper health care due to erroneous data.
Professor Peter Colvonen, UC San Diego, noticed discrepancies in the data that was collected when he was working on a consumer wearable sleep study. Fitness trackers were more accurately able to detect a heart murmur and hypoxia for white people than black people. The high error rates and gaps in readings were all from African Americans[3]. Another example of bias in wearables.
Medical-grade respirator masks offer protection for health workers if they fit properly. As the mask is a one-size-fits-all garment, calibrated to white males, there have been issues with protection for female wearers as well as certain ethnic minority groups[4].
Experts also believe there are racial biases in the interpretation of data gathered from spirometers, measuring lung capacity4. There is a working assumption (possibly inaccurate) that ethnic minorities have a lower lung capacity than white people. Due to the lower baseline lung capacity for ethnic minorities, when a white and non-white person records the same lung capacity via a spirometer before adjustment, the white person is being prioritised for treatment after adjustment for the baseline.
Gender bias may exist too in medical settings in Australia. A 2018 study published in the Medical Journal of Australia[5] showed six months after hospital discharge, death rates and serious adverse cardiovascular events in women presenting with ST-Elevation Myocardial Infarction (STEMI) in the past decade were more than double the rates seen in men.
“The reasons for the under-treatment and management of women compared to men aren’t clear,” the study states. “It might be due to poor awareness that women with STEMI are generally at higher risk, or by a preference for subjectively assessing risk rather than applying more reliable, objective risk prediction tools.”
These are just a selection of areas where medicine is currently exhibiting bias and the insurance industry needs to be involved in advocating for the reduction of these biases hence ensuring the medical data they use is accurate and informative.
Final thoughts
Even though implicit bias has been widely discussed for many years now, digitalisation is speeding up the use of home devices to assess our medical condition. As measurements from these devices are shared to use in medical studies, in the underwriting process and other areas, these biases need to be recognised and adjusted for and then the root cause addressed and eliminated.
The first step is raising awareness, so people robustly question the level of implicit biases when designing products and services and using datasets derived from biased sources. Secondly, we need to establish ways of testing for these biases. We will need to create dedicated roles within larger organisations, to seek out implicit biases and propose solutions. Additionally, in order for this to become part of the regular workflow within organisations, there will need to be incentives to find and address these biases or penalties for failing to do so, thus ensuring fairness permeates through every part of our systems. A robust dialogue on this issue is a good starting point.
References
|
CPD: Actuaries Institute Members can claim two CPD points for every hour of reading articles on Actuaries Digital.