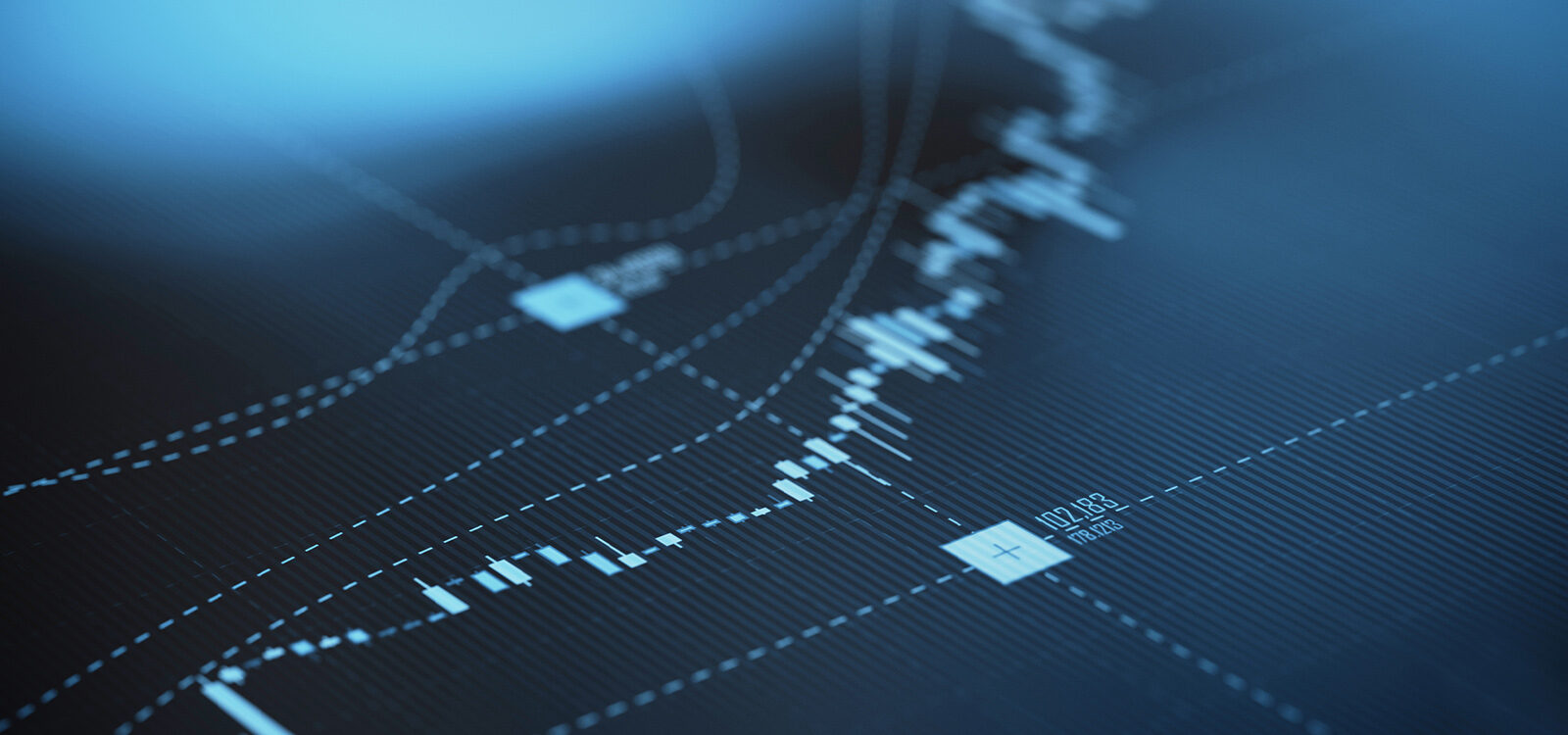
The privacy act is changing – how will this affect your machine learning models and what can you do?
In a world increasingly tied to artificial intelligence and machine learning, Taylor Fry’s Jonathan Cohen and Stephanie Russell look at changes to the Australian privacy act currently under consideration and how to address the potential impacts for industry and consumers.
Community concerns have become more urgent in recent years regarding the way businesses collect, use and store people’s personal information. In response to a recommendation from the Australian Competition and Consumer Commission’s (ACCC) Digital Platforms Inquiry (DPI) – the Australian Government is reviewing the Privacy Act 1988 to ensure privacy settings empower consumers, protect their data and best serve the Australian economy.
Of the several proposed legislative changes, we outline three scenarios being considered and how they may impact organisations that collect and process customer data – in particular, those organisations that use machine learning algorithms in privacy-sensitive applications, such as facial recognition. We break down the three proposed changes below:
- Expansion of the definition of personal information to include technical data and ‘inferred’ data
- Introduction of a ‘right to erasure’ under which entities are required to erase the personal information of consumers at their request
- Strengthening of consent requirements through pro-consumer defaults, where entities are permitted to collect and use information only from consumers who have opted in.
1. Treating inferred data as personal information
The Government is considering expanding the definition of personal information to also provide protection for the following:
- Technical data, which are online identifiers that can be used to identify an individual such as IP addresses and location data.
- Inferred data, which is personal information revealed from the collation of information from multiple sources. For example, an analytics company may combine information about an individual’s activity on digital platforms, such as interactions and likes, with data from ‘smart’ devices to reveal information about an individual’s health or political affiliations.
The possibility of including inferred data represents a fundamental expansion in what organisations might think of as personal information. Personal information is typically information provided by a user that is known with a reasonable amount of certainty. In contrast, inferred information can usually only ever be known probabilistically. Under the proposed change, knowledge such as ‘there is an 80% probability that this customer is between 35 and 40’ could be treated the same way as knowledge such as ‘this customer is 37’.
This means model outputs may become ensnared in restrictive governance requirements because inferred customer information is often generated as an output of machine learning models. There is even a possibility that governance requirements may be extended to the models themselves, which have been shown to leak specific private information in the training data to a malicious attacker. The consequences for machine learning model regulation are potentially hugely significant, given personal information is subject to many more rights and obligations than the limited set currently applicable to models.
Newly afforded rights for consumers may mean that they can request information on model origination, and restrict future processing and use of models. On top of this, companies may now be required to design models that comply with data protection and security principles, and to discard models in order to comply with storage limitation principles.
2. Right to ‘erasure’ – deleting personal information by customer request
Put into effect across the European Union in 2018, the broad ranging General Data Protection Regulations include a ‘right to erasure’, which provides citizens with a right to have their personal data erased under certain circumstances, including when consumers have withdrawn their consent or where it is no longer necessary for the purpose for which it was originally collected. This serves as a reference point in the Australian Government’s review of privacy laws.
Given machine learning models can be considered as having processed personal information, a consumer may wish to exercise their right to erase themselves from a model to remove unwanted insights about themselves, or to delete information that may be compromised in data breaches.
In most circumstances, the removal of a single customer’s data is unlikely to have a material influence on a model’s structure. The ‘right to erasure’ becomes more powerful when exercised collectively through a co-ordinated action by a group of related customers, as their data is more likely to have had a material influence. For example, the use of facial recognition technology for identifying a marginalised group may cause a collection of customers to feel they are disadvantaged by the ongoing use of models trained on their data.
However, it is plausible that even an erasure request from a single customer would require the removal of their data from the model, as well as their exclusion in data processing. This would be a very tall order for organisations to comply with, to the point of being unworkable in some situations where the cost and time required to comply with erasure requests rapidly outweighs the benefits of using machine learning models.
3. Strengthening consent – how will it affect model insights?
With consumers preferring digital platforms only collect the information they need to provide their products or services, the final ACCC Digital Platforms Inquiry report recommended that default settings enabling data processing for purposes other than contract performance should be pre-selected to off, otherwise known as ‘pro-consumer defaults’. This echoes some changes occurring internationally, such as Apple’s decision to require opt-in for apps to track consumers across multiple apps and websites.
The potential implication of pro-consumer defaults is that the data available to organisations for future training of machine learning models may be limited, as it can be reasonably expected that relatively few consumers would make the effort to deliberately reverse these settings.
Consider an extreme scenario, whereby historical data that was collected prior to the introduction of the laws is no longer permitted to be used. Many organisations may struggle in the weeks immediately following the change, and could effectively be required to rebuild models from scratch at a time when very little data may be available.
Assessing your privacy risks – steps you can take now
With the review still underway, the final form of the changes made to the privacy act remain uncertain, with the potential ramifications for machine learning models even more so.
Nevertheless, there are some practical steps organisations can take now to assess and reduce potential privacy implications for their machine learning models and pipelines:
- Establish or review the composition of a cross-functional data working group to understand and respond to compliance and governance requirements
- Review data usage policies and have a clear understanding of existing customer consents
- Track and document the use of customer data in models, including the use of technical and inferred data.
Taking these steps will provide organisations with a good view of where privacy risks may arise, and ensures they are better prepared for the potential implications of changes arising from the review of the privacy act.
This is an edited version of an original article published by Taylor Fry. |
CPD: Actuaries Institute Members can claim two CPD points for every hour of reading articles on Actuaries Digital.