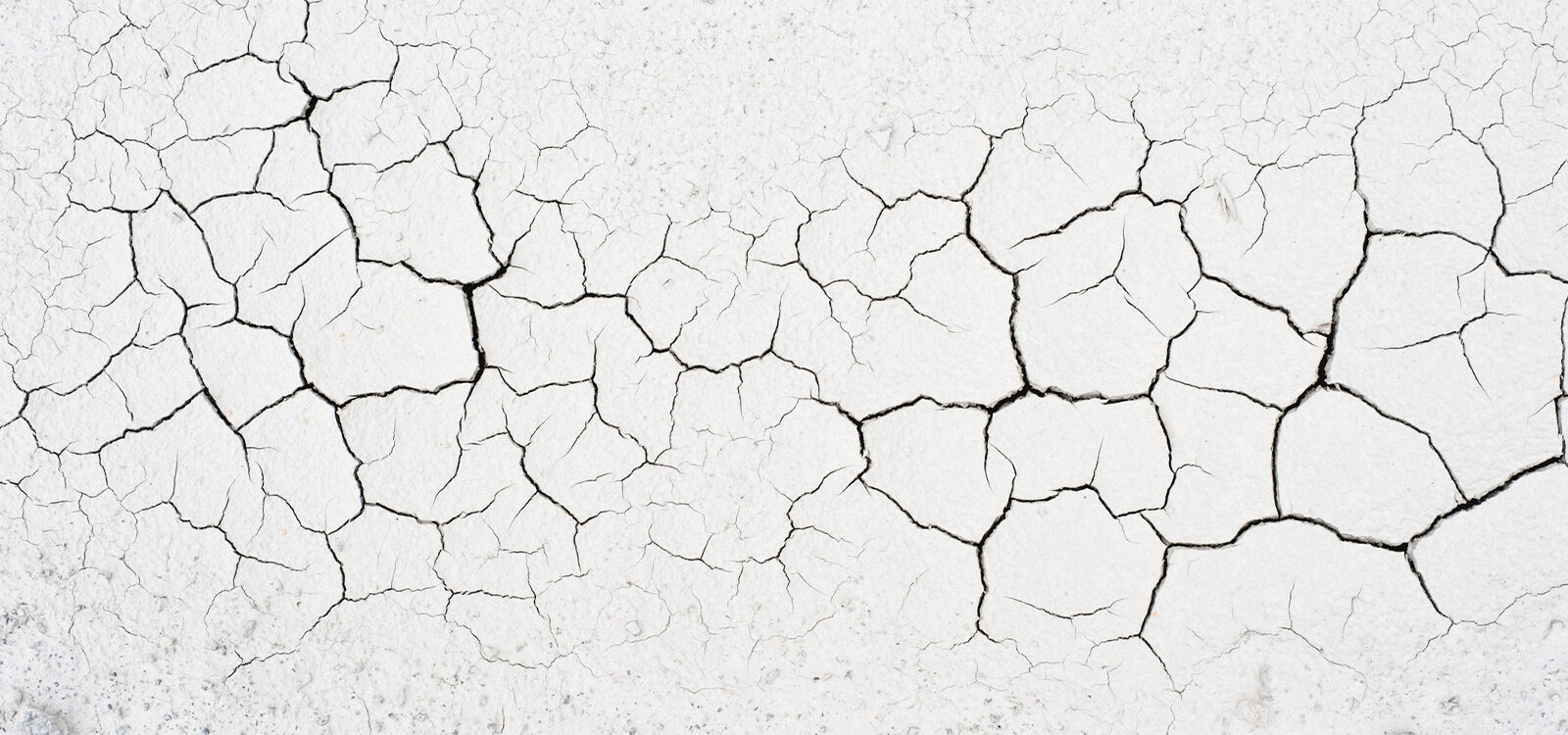
Making sense of physical climate risk assessments
The sudden surge in demand for climate risk insights within the corporate sector has led to a corresponding proliferation of climate service providers (CSP). However, given the inherent complexity of climate risk, subscribers of the service find themselves “drowning in information yet starving for knowledge” – potentially leading to dangerous misinterpretation of CSP results. Alex Pui and Neil Aellen shed light on the key uncertainties within the climate modelling process and offer suggestions on how corporates can better make sense of physical climate risk assessments.
Following the stark warning from the consortium of central banks to corporates to ‘adapt or fail to exist in the new world’ in 2019, corporate momentum has been steadily building within the climate risk disclosures and broader sustainability space.
Due to the inherent complexity of the subject matter, and lack of in-house expertise across most corporates, there has been a recent proliferation of CSPs’ to rapidly growing market demand, particularly within the financial sector, where the potential for impacts from more frequent and severe climate catastrophes pose systemic financial risk.
As climate risk disclosure standards continue to be refined through the Network for Greening the Financial System (NGFS) and Task Force on Climate-related Financial Disclosures (TCFD) initiatives, the vast information asymmetry between subscribers and providers of climate risk services has emerged as a risk in itself – the risk of misinterpretation and overreliance on CSP-generated insights[1].
As such, this article proposes how firms can make sense of physical climate risk assessments namely by striking a balance between excessive academic rigor on one hand, and guarding against the risk of misinterpreting potentially spurious model results to ultimately help corporates extract meaningful value from climate risk analysis.
Understanding cascading uncertainties introduced in the climate modelling chain is key
Due to the gravity of business and policy decisions stemming from climate risk analysis, the real starting point for engagement goes beyond applying CSP output to inform business or risk management strategy, but first building a keen appreciation of the capabilities and limitations of the climate modelling chain (see schematic 1)[i].
Key sources of GCM uncertainty
At the start of all chains lies Global Climate Models (GCMs). These resolve thousands of physical equations that represent our current best understanding of the climate system, and are then used to reproduce the past, and project future climate conditions – such as long-term average and extreme precipitation and temperature statistics in a future reality – as opposed to precise weather conditions days to weeks forward. Although highly sophisticated and powerful, GCM projections fundamentally contain three main uncertainties:
- emission scenario uncertainty (trajectory of GHG emissions based on socio-economic developments and policy decisions);
- model response uncertainty (divergent model views based on the same emission scenario due to assumptions/parameterisations used to compensate for incomplete knowledge of exact physical processes, GCM resolution); and
- Natural internal variability (inherent uncertainty or ‘noise’ based on periodic low frequency climate modes such as El Nino Southern Oscillation phenomena)
Emissions scenario uncertainty is represented through the use of different representative concentration pathways (RCP), or more recently shared socioeconomic pathways (SSP) scenarios, while internal variability and model uncertainty is best reduced by using long control simulations and relying on a projections from a variety rather than single GCM (called ‘ensembles’). While GCM skill is determined based on how faithfully it reproduces historical statistics, or to what extent different GCMs ‘agree’ with each other in the projection of various climate variables, this does not necessarily guarantee robustness for future projections. For example, will established physical relationships in the past continue to hold into the future?
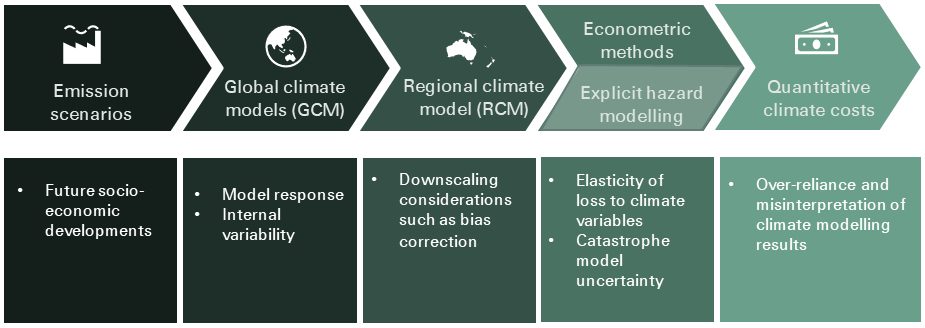
Post-processing of GCM outputs: Downscaling and Bias Correction
As raw GCM outputs are often too coarse in space (>100km) and time for a range of more localized applications such as developing future flood risk profiles, the next step in the modelling chain involves ‘downscaling’ either using dynamical (via running regional climate models RCMs at a higher resolution constrained by boundary conditions established by the GCM) or statistical means which leverage established historical links between local and large scale climate observations. Depending on which method is deployed, systematic model errors or biases are introduced due to uncertainties in observational datasets which downscaling heavily relies upon. For RCM based downscaling, bias correction is required to identify and close systematic ‘gaps’ between observed and simulated climate variables over available historical period by way of a ‘correction factor’. Although this ‘correction factor’ assumes that biases in the future mimic the past, bias correction is an important step in creating more realistic absolute climate projections as driving data for applications down the modelling chain such as hydrological models.
‘Top Down’ vs ‘Bottom Up’: which is better?
The next stages of the modelling chain diverge significantly with two broad approaches undertaken to produce quantitative estimates of climate risk: namely, top down macroeconomic approaches incorporating the elasticity of productivity and other variables to rising temperatures as well as ‘bottom up’ methods which involve specific hazard and catastrophe modelling. While these two approaches are not directly comparable as they are underpinned by completely different methodologies, it is arguable that the macroeconomic approach based on disaggregating GDP by industry sector is more appropriate for large sectoral scale analysis of chronic, rather than acute climate risk. Here, the assumptions made while establishing the tenuous link between regional average temperature projections and sectoral based loss estimates is primarily where uncertainty is introduced.
Conversely, ‘bottom up’ approaches allow the incorporation of specific climate peril risk drivers, such as flood and storm impacts on various building types through tractable catastrophe modelling frameworks which has been used extensively by insurance companies for several decades in existing property underwriting analysis. Through careful adjustment of relevant parameters to reflect climate change impacts, the hazard module of catastrophe models which includes stochastic simulations based on historical observations, can be adapted to reflect future hazard risk profiles. Although catastrophe models also face challenges particularly in estimating complex supply chain induced business interruption losses, it enables more transparent scenario stress testing in the adjustment of hazard and building vulnerability parameters (for example to cater for anticipated improvement in building design standards) and can also be used for sensitivity testing of risk mitigation measures at local or regional scale.
Linking physical climate risk insights to tangible business outcomes
Linking climate risk insights to business outcomes is the next critical step. The sheer breadth of the corporate landscape compels tailored rather than a ‘one size fits all’ solution. For example, while banks, insurers and large manufacturers may all be interested in the future evolution of flood risk, all three groups have different key applications. Banks and insurers would be more concerned regarding the longer term impact of flood on broad scale mortgage default probability (as at time of maturity several decades later) and increased claims burden and premium inadequacy issues respectively, while a manufacturers’ key focus is likely on identifying key critical weaknesses in its complex global supply chains.
Flood aside, sectors such as energy transmitters are increasingly wary of other perils such as wildfire, where recent large fire events in California and South East Australia have resulted in and sudden rate hikes and tightening of capacity. These are but a few of the myriad applications leveraging climate risk insights, and while each application will be distinct, we propose the following key considerations for corporates undertaking climate risk assessments:
Internal climate risk education
Placing an emphasis on internal climate education and capability build forms a natural first step especially as the core business of most corporates is not within a climate related field. While large sections of climate risk assessment may still be best outsourced to specialist providers, it is important for subscribers of the service to be in an informed position to critically assess the quality of climate service provider outputs. Technical specialists aside, improved awareness at a senior management level will not only help firms better navigate broader sustainability strategy, but also guard against risk of greenwash or misinterpretation of climate risk insights which could lead to poor business decisions.
What are the corporate(s) key goals of climate risk assessment?
Establishing clarity around the motivations for performing climate risk assessments will help achieve the right balance between level of complexity and lead to more focused outcomes – such as responding to regulatory requirements (for relevant financial sectors), risk management and future business strategy considerations. However, for some firms, exploratory climate risk analysis with less stringent timelines may be preferred for firms just starting out on climate risk assessment journey and should be explicitly communicated as such to manage key stakeholder expectations. Irrespective of motivation, climate impact assessment is a long and iterative journey so building a flexible framework that allows monitoring and convenient updates when new risk views become available is a worthwhile investment.
Supplementing model output with holistic view of risk
There have been many prominent examples of over-reliance on model outputs, notably the failure to capture tail dependence in copula driven financial models during the GFC in 2008, or the US election outcome of 2016 where models missed the strong correlation amongst states within the rustbelt. In the context of climate assessment, key model assumptions and results need to be sense checked such that a broader and more nuanced view of risk can be achieved. For example, examining historical observed climate trends, and particularly for acute climate risk – accounting for near misses (or counterfactual analysis). As models are often trained upon high quality, but relatively short observational data sets, future climate risk insights could also be compared to paleo data when developing realistic loss scenarios. Lastly, as COVID-19 has painfully laid bare, corporates need to critically consider a range of modelled outcomes rather than a single point estimate. This is important as it accounts for non-modelled inter dependencies between compound events such as climate disasters occurring during a time of systemic compromised economic resilience during a global pandemic.
CPD: Actuaries Institute Members can claim two CPD points for every hour of reading articles on Actuaries Digital.