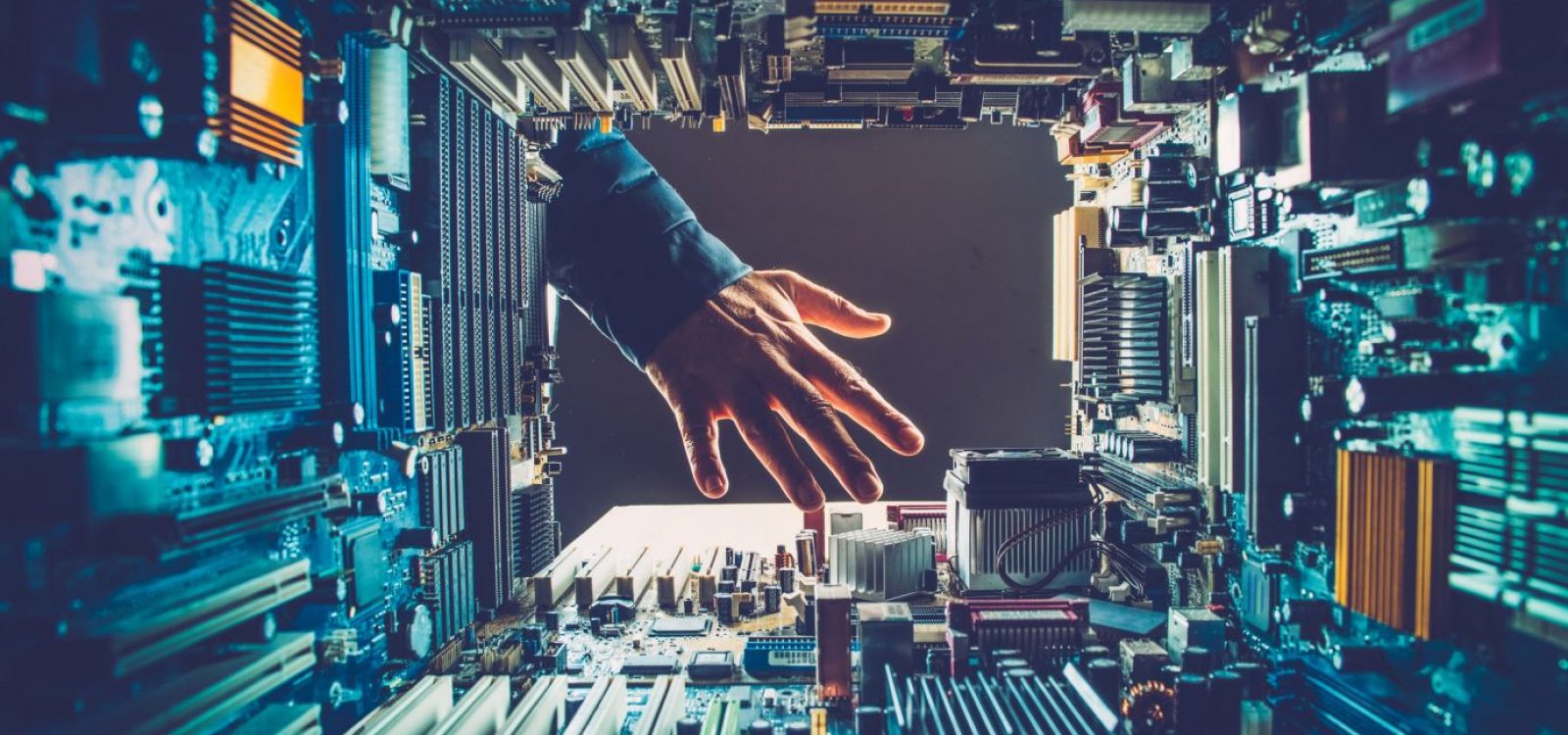
Normal Deviance – A Tale of two CT(O)s
A big data parable, wherein Alice learns steady steps and Bob aims big.
Alice is the chief technology officer (CTO) of a medium-sized financial services company. She’s been asked to lead a one-year project to enhance the business as part of the company’s wider ‘big data’ push. While a bit daunting, she’s accepted that there is plenty of potential. After a quick brainstorming session, she’s picked three specific initiatives that should add some value in different areas of the business: a targeted customer retention initiative, a price elasticity exercise and a project around call-centre satisfaction scores.
Bob is also a CTO of a similar company and has been given a similar brief. Bob is delighted to be given such a high-profile piece of work. Initial meetings with a wide range of internal stakeholders give him further confidence – all parties are excited by big data opportunities, particularly given the extra budget set aside for the work. The project is positioned as a whole-of-company change initiative, with metrics around increased revenue and profitability across all product lines.
Alice consults with the data warehouse team. They have been working hard for the past few years improving the accuracy and timeliness of important company datasets. This work proves invaluable; there are already relevant and rich datasets related to customer retention and call-centre satisfaction. However, there is not much information on price-elasticity. Alice adjusts the price-elasticity initiative so that the main objective for the year is a new dataset that will form the basis of future work. A team of modellers begin work on the other two initiatives, with a view to finding some target customer groups for intervention.
Bob talks to his modelling team about how they can use their big data. One analyst identifies a couple of datasets that haven’t been used for much analysis yet – one relates to customers upgrading their products and the other a collection of competitor prices. Bob think these sound great, and asks the team to do some modelling and report back with some insights.
While modelling goes on, Alice talks to the customer loyalty team and call-centre managers about their thoughts for strategic improvement. The loyalty team have been considering a range of targeted offers, but haven’t had information about how generous the offer should be, or which customers to target. When the modelling team reports back, they identify six distinct segments with high risk of leaving. Alice does not have an immediate feel for which targeted offers will work best, so decides to try three different offers for each segment (plus keeping a control group within each segment). Budget is approved and the trial is quickly rolled out. The call-centre similarly has some ideas; staff are trained to respond to a specific complaint in three different ways and data systems set up so the difference can be measured.
Bob’s modelling team reports back and shows there are lots of significant effects. Older customers are much more likely to upgrade, as are those from high socio-economic areas. One executive asks whether this means they should advertise more to high socio-economic customers or lower ones. The modelling team aren’t sure; the data doesn’t really answer that question. They’ve also discovered insights from the competitor price data; when their competitor reduced prices there was a measurable decrease in new customers coming from their competitor. Again, they couldn’t say whether this meant targeted price reductions would be effective. Bob’s excited by the findings and decides to workshop them more broadly in the company.
Alice now has early results from both initiatives (and good progress on the new elasticity dataset). Two of the targeted offers are cost-benefit positive, and appear most effective on different segments. They are rolled out more broadly, while maintaining a control group for baseline comparison. One of the call-centre approaches is also materially improving customer satisfaction and that becomes the new default training for all staff dealing with that complaint. Specific metrics are measured around customer retention (and associated revenue gains), while call centre customer satisfaction was already a tracked metric.
After a month organising a meeting with key executives, Bob presents the results of the insight models. Executives are impressed enough to renew funding for another year. One executive asks how revenue improvement will be modelled, and Bob agrees that it would be good to add this to the plan. There’s a long discussion about what operational responses should be, but ultimately the decision is deferred until there’s more information. It’s also too early to see promised improvements to revenue and profit.
Alice and Bob are both onstage in a panel discussion at an industry conference at the end of the year. They both espouse the virtues of big data analytics and its potential to improve company performance. However, Bob can’t help but shake the feeling that maybe he’s not doing it right…
The 2017 Data Analytics Seminar looks beyond the data science bubble to address how you can utilise data analytics to deliver real value. View the Program and Register Now here.
CPD: Actuaries Institute Members can claim two CPD points for every hour of reading articles on Actuaries Digital.