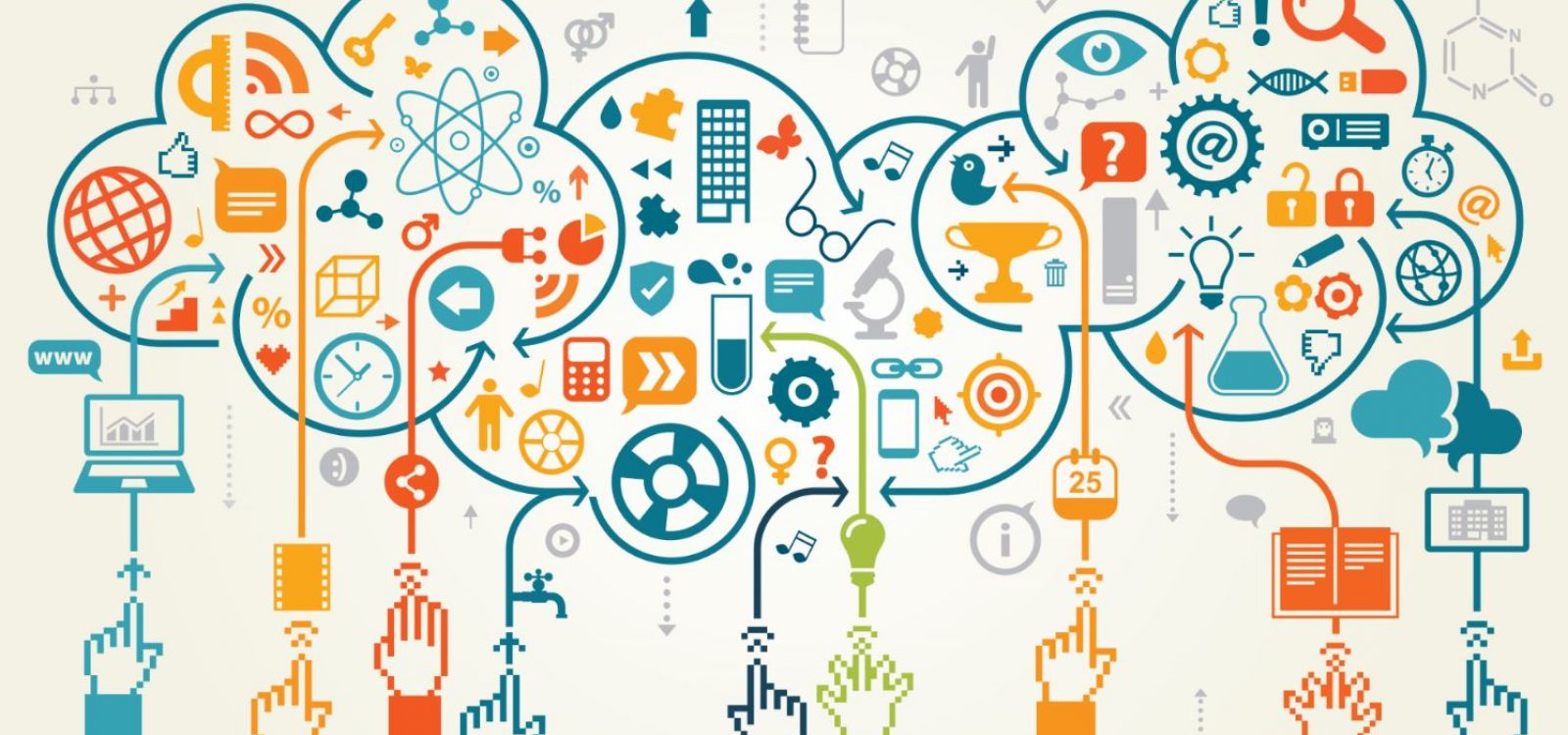
DAT203x: Data Science and Machine Learning
How long has the concept of Data Science been around and what are the best tools available for mining ‘big data’? A new MOOC coordinated by the Actuaries Institute’s Data Analytics Working Group presented the latest knowledge in this exciting field. Course participant Kriti Khullar AIAA, reports.
During my participation in the Data Science and Machine Learning MOOC (Massive Open Online Course) I discovered that as early as 1996 the concept of data science and the buzzword ‘big data’, was presented via the KDD (Knowledge Discovery in Databases) process (see below). I was surprised to see that Data Mining was present back then!
Then in 2000 emerged the CRISP –DM (Cross Industry Standard Process for Data Mining) Process:
More recently, the CCC Big Data Pipeline came out in 2012:
Our lecturers Cynthia Rudin (Associate Professor of Statistics at MIT) and Steve Elston (Co-Founder & Principle Consultant of Quantia Analytics) thought that these processes were similar, but written in different contexts and with different purposes. They inherently capture the simple statement:
Data is selected (based on business needs and target audience), it is then cleansed and prepared, then subsequently transformed for modelling and/or data mining purposes, before it is meaningfully interpreted through statistical means and applied for more informed decision making and subsequently, actionable results are formulated, creating create value add information.
This is essentially what Data Science is about. Of course, it is an iterative process with data cleansing and transformation being accountable for 99% of the work but this is what assists in meaningful results and better-informed decision making. This can also be observed in the below graphic.
Amanda Aitken, member of the Data Analytics Working Group, participated and answered the many questions that were raised in the study groups. Questions were raised by curious cats around what is the best tool out there for visualisation? Is it the Microsoft Azure Studio or is it Tableau or is it QlikView? (I wonder what you use as a reader – consider sharing as a comment on this article below!) Discussions arose around the limitations of each of these tools. It was also great that when one student shared a problem, it wasn’t just Amanda who assisted in its understanding and resolution but also other course participants as well.
Having the Microsoft Azure Learning Studio with its own Data Library was also quite useful in providing participants with a hands-on experience alongside the theoretical learning of scaling, normalising the data, truncating the data and the use of the metadata editor (and a lot of more of course). It was useful navigating through the variables in these datasets and the distribution curves associated with these variables as can be observed in the examples below.
Example 1: Distribution of variables in Microsoft Azure
Example 2: Gooey interface of Microsoft Azure
This Data Science and Machine Learning can be applied in several facets of the banking industry via the collection and analysis of Big Data to enable better identification of customer needs and subsequently more targeted marketing. This may involve adopting a big picture approach to identify business opportunities by observing how customer relationships may be interconnected, i.e. a say there is a plumber who is a customer of the bank MNO but at the same time, the plumber’s company XYZ may also be a customer of the bank MNO. Developing a model to capture this relation (amongst others) combined with analytics can be conducted to identify patterns and provide a more efficient and needs-based solution to the customer.
Of course, one of the obstacles for using data science in a banking industry is the legacy platforms and databases that exist and the idea of placing confidential information on a cloud based service. Nonetheless, there is too much data out there that can be used and the beauty of Data Science is that it allows data visualisation to simplify the most complex piece of information to something which can be read by non – technical experts to drive business strategies. This could transform the entire banking industry just like how the passbooks were replaced by cards. In the same way with the assistance of Data Science, block chains could be used to create smart contracts. But that discussion is for another day. I wonder what the next study group will cover…
The DAWG will be running a second MOOC study group in September 16. Details of the MOOC to be studied can be found here. For more information, or to register for the study group, send your full name and preferred e-mail address to education@actuaries.asn.au.
CPD: Actuaries Institute Members can claim two CPD points for every hour of reading articles on Actuaries Digital.