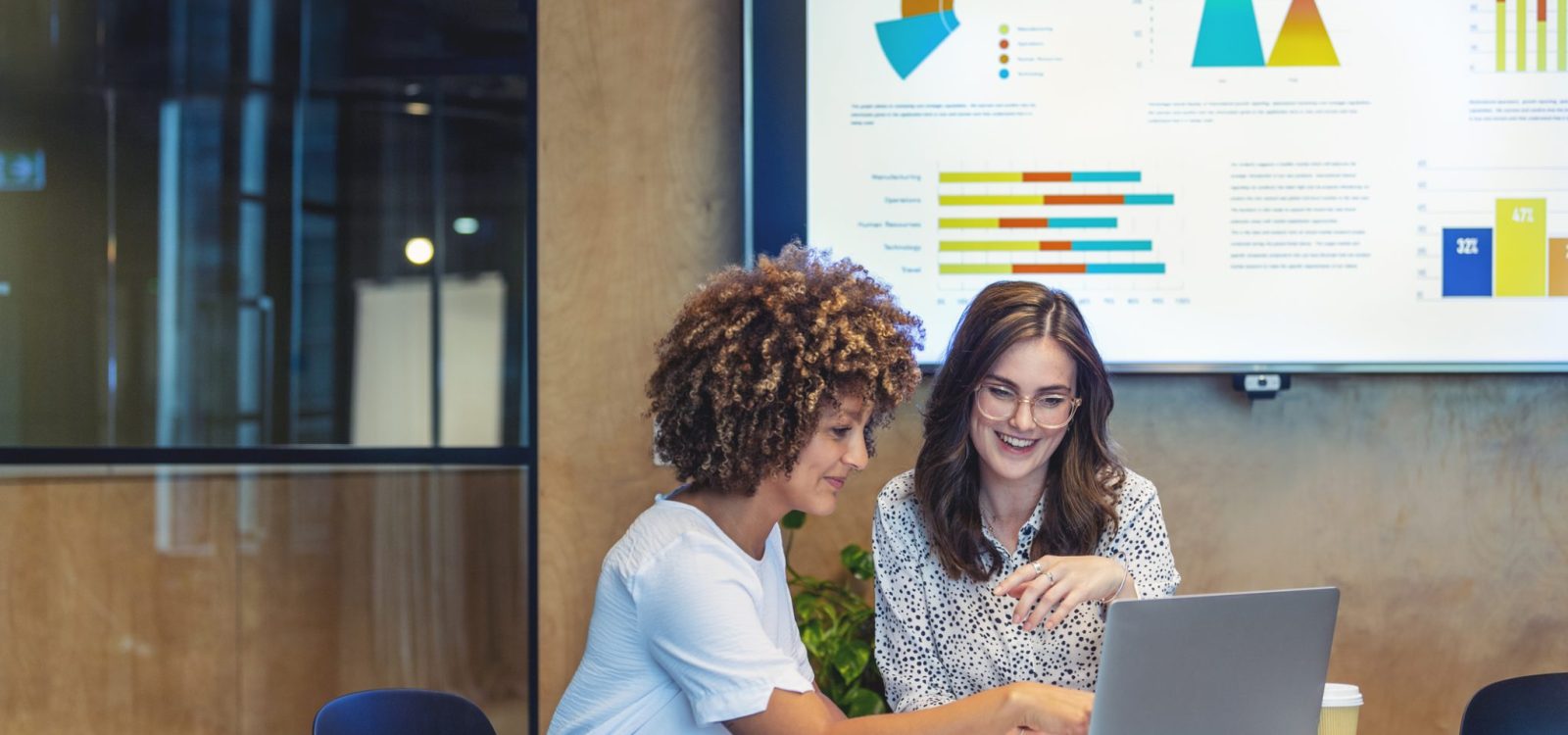
Finding the Common Threads
In this month’s Normal Deviance column, Hugh reflects on how similar ideas arise in different professions, and what this says about work and education.
I like to spend a lot of time reading the work of other people – albeit never as much as I’d like. Our ability to add value in the world relies on staying up-to-date with progress, and this is certainly true for applying data analytics.
When doing some background reading, sometimes I’ll find a piece of knowledge that is genuinely new to me – a new model idea, or a new subject area. However, increasingly I am also appreciating the overlaps with existing knowledge as I read. This is when ideas can be readily related to others. In such cases, identifying the common thread and understanding the cohesion is both valuable and satisfying.
Sometimes the cohesion occurs in very technical domains – for instance, I wrote a Paper in 2015 exploring the pretty fundamental connections between penalised regression, credibility models, and boosting. And I still find the generalised linear models as a useful paradigm for comparing new prediction models, too.
More generally, it seems to happen most when moving across different domains of academic literature – say, between economics, statistics, actuarial and social sciences. Parts of research that have evolved separately will still carry common ideas or approaches that can be synthesised.
That feeling of pulling together related threads has happened to me a few times recently beyond technical areas, and I wanted to share a couple of examples.
The Control Cycle
Actuaries (rightly) take pride in the control cycle, which is a core part of our syllabus. While there are variants, the basic control cycle steps are to define the problem, design a solution and monitor results. This cycle is then covered by our professionalism and subject to impact from external forces.
Figure 1 – The actuarial control cycle, as it was taught to me
While actuaries are probably not the inventors of the first ever cycle, it is longstanding and enduring. And what is fun is to keep an eye out for how control cycle thinking arises in other places over time, in different areas of work and study. The risk management control cycle is an obvious one, but in more recent times I’ve spotted the following:
- Many statistics courses now teach the PPDAC cycle of investigation as a way of framing a statistical analysis, which (I think) has grown out of New Zealand (NZ) statistical education and was advocated for in David Spiegelhalter’s excellent 2020 book.
- As the need for AI governance has risen over time, people have proposed management cycles for their governance. Picture below borrowed from Collibra.
- Policy and evaluation has a similar dynamic to actuarial, often characterised as a cycle.
- Perhaps most delightfully, I came across a dental control cycle, which is strikingly similar to the actuarial one.
Figure 2 – Cycles, cycles everywhere
What do we make of the plethora of cycles? Probably not too much. Clearly, the actuarial control cycle is tapping into something fairly universal and profound – that a piece of work (actuarial or otherwise) benefits from good planning, delivery and ongoing monitoring. Perhaps it takes away a little of the uniqueness of the actuarial model but, on the upside, it confirms the actuarial mindset is well-equipped to tackle issues more broadly.
Microsimulation
A second example is microsimulation. One of the first major government projects I was involved in was the Investment Approach Valuations of the NZ welfare system. The methodology was largely designed from scratch, but was an individual-level model that focused on transitions between different welfare benefits. The method proved useful, giving detailed individual-level and cohort-level insights as well as top-level control. Since that time, quite a few similar ‘investment approach’ simulation models have been developed across different sectors such as justice, housing and child protection.
What I didn’t appreciate fully (mainly due to my naivety at that point in time) is that we’d stumbled into quite a large world of microsimulation. Discovering other related work, and different approaches and paradigms, has been pleasing. To give some examples:
- Academic microsimulation models have existed in various forms over time. NATSEM at the University of Canberra was a good early example of this, particularly their STINMOD model for tax and transfers. Some related microsimulation work is now done by Commonwealth Treasury internally.
- Agent-based models have grown in popularity. These have similarities to microsimulations, but often emphasise the connections between different people. For example, agent-based models of COVID-19 spread emerged as an alternative analysis tool to traditional dynamic models of infection.
- Areas like transport and traffic modelling have long used microsimulation to better understand the impact of tweaks to the road and greater transport system. Modelling sophistication is pretty mature, with many specific software solutions.
Final thoughts
As knowledge continues to bifurcate, being able to see the overlaps can be as useful as learning new things. This makes it easier to build on existing knowledge and learn from situations where similar ideas and problems have arisen. I think this represents both an encouragement and a challenge as actuaries continue to explore new domains.
CPD: Actuaries Institute Members can claim two CPD points for every hour of reading articles on Actuaries Digital.