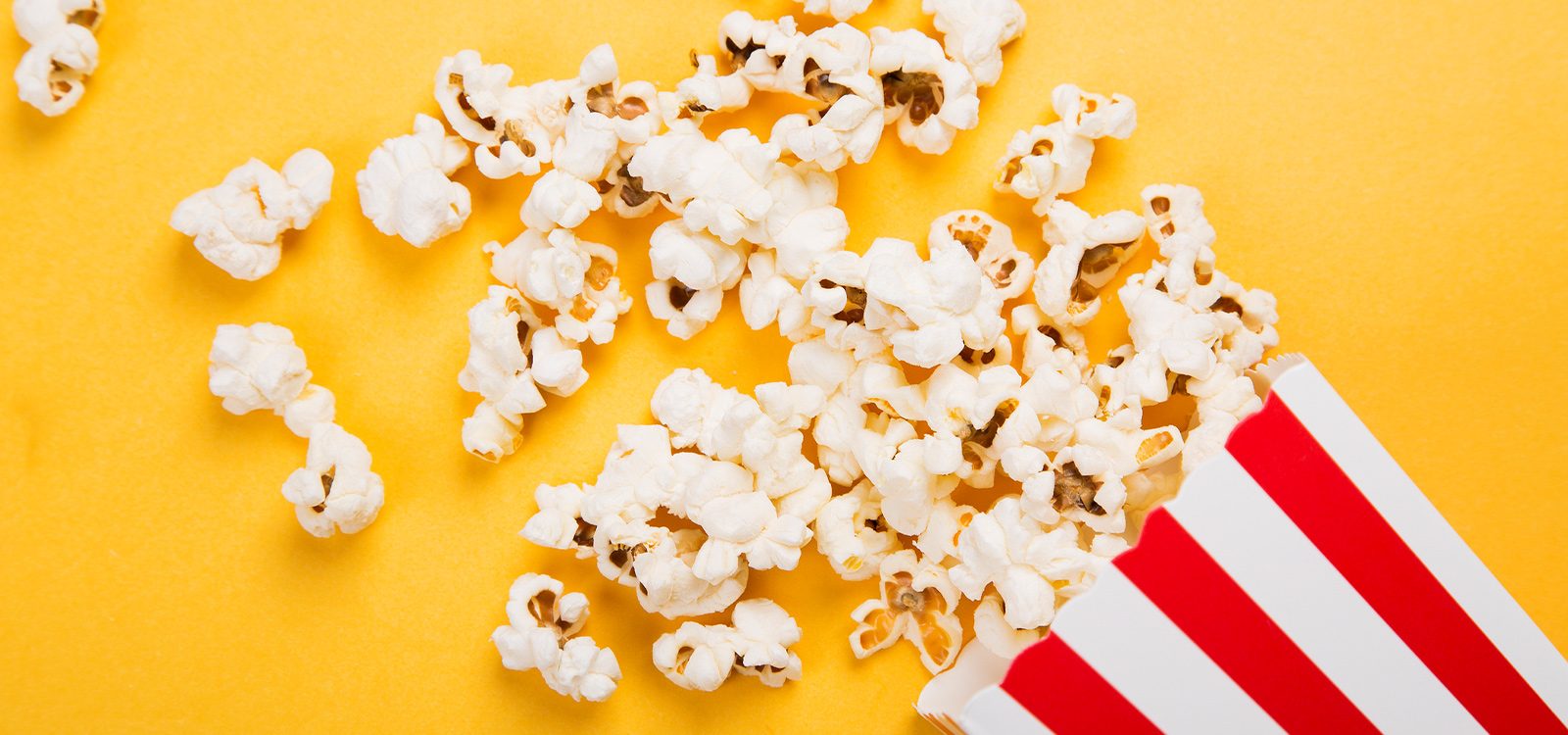
‘YDAWG Analytica’ go to the movies
We at the Young Data Analytics Working Group (YDAWG) are used to looking at the world through a different, data-focused lens. So, despite the millions of different articles, opinions, and hot takes from this week’s Oscars, we have managed to put together a unique and interesting way to look at some of the greatest movies from recent history. So, pop your favourite popcorn, import your favourite data packages, and prepare yourselves for another ‘YDAWG Analytica’ article!
In this article, we use Data AnalyticsTM to look at:
|
Natural subtitle processing
In this analysis, we primarily used the NLP technique of ‘sentiment analysis’. This is a technique that takes a group of words or sentences and uses a language model to measure the polarity (whether the opinion is positive/negative) and intensity (strength between 0 and 1).
We’re using a popular python package called NLTK and its pre-trained sentiment lexicon called Valence Aware Dictionary for sEntiment Reasoning or ‘VADER’ for short (conveniently fitting in with our movie theme). Often, this is used for reviews and testimonials to gain more insight into customer satisfaction.

This technique can be used on individual words, sentences or whole comments. I’ve picked out how VADER rates a few specific words, so you can see that the sentiments for individual words line up with human expectations (except personally I would rate the word data as highly positive!). Most words end up neutral, but the model is usually pretty smart about how it understands groups of sentences as a whole.
So, we can train computers to understand reviews, but how about movies? To attempt this, we came up with a novel and creative solution to use NLP on subtitles and track sentiment over time!
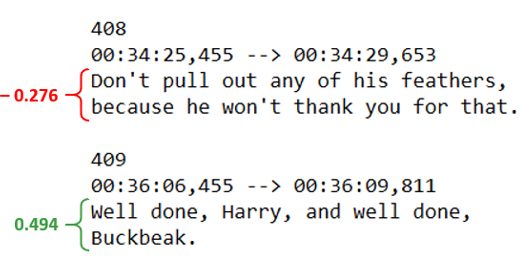
We conveniently have timestamps with every piece of dialogue too, which means we can analyse these subtitles as a time series. With a little bit of python magic, we can add an aggregation function for a rolling 10 minutes of screen time to produce graphs like this.
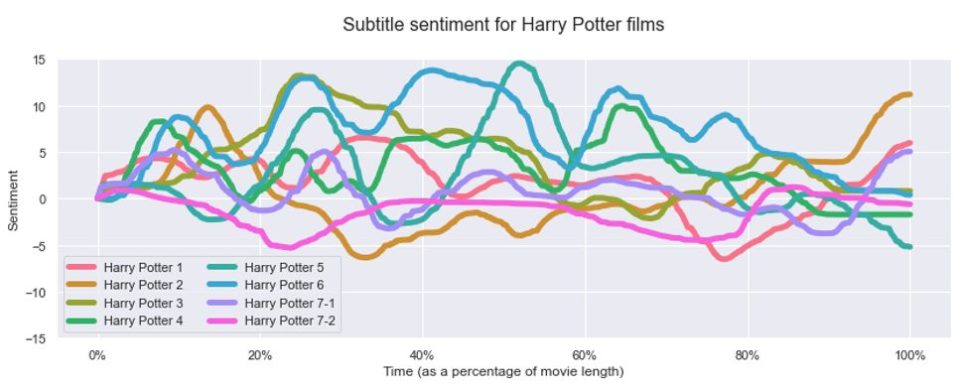
A (best) picture is worth a thousand (best) words…
While a picture can tell a thousand words, it’s often easier to just use a thousand words to give us a better picture. So, let’s use our newfound NLP skills to analyse all of the Best Picture nominees for the past few years of the Oscars.
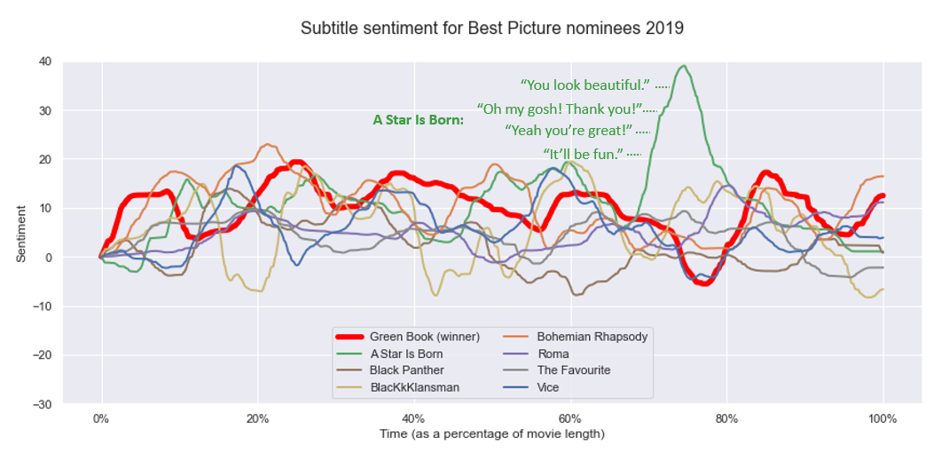
On each graph, we have highlighted the winning movie in red, which for 2019 was Green Book. You can see the peak for one of the nominees, A Star Is Born, comes from a long series of positive compliments and remarks in a short window. There is even a celebratory montage and accompanying positive song lyrics that help it reach these high heights.
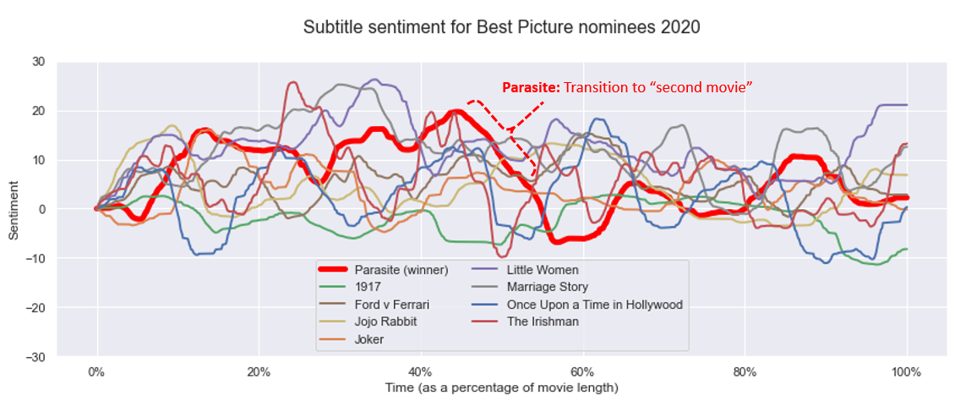
Parasite, Best Picture winner for 2020, has been hailed as a masterpiece that upends the familiar ‘three-act’ structure that we are used to. Instead, the film is almost two separate movies joined into one. In the graph above you can see that our NLP analysis is able to pick out when exactly the transition sequence kicks off and we move into the ‘second’ movie.
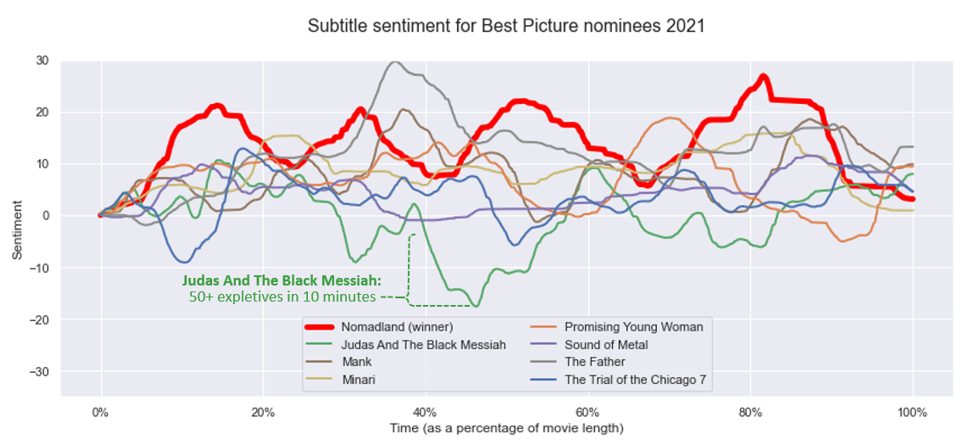
As you might imagine, there are sometimes key phrases or words which are interpreted by the model as especially negative or positive in every context. In Judas and the Black Messiah, an already dark movie, you can clearly see a portion where there are a lot of expletives used. While some of these quotes and text blocks are actually using these swear words in a neutral or positive way, the large majority of the time this is not the case. Either way, we won’t be including any of these quotes in this article.
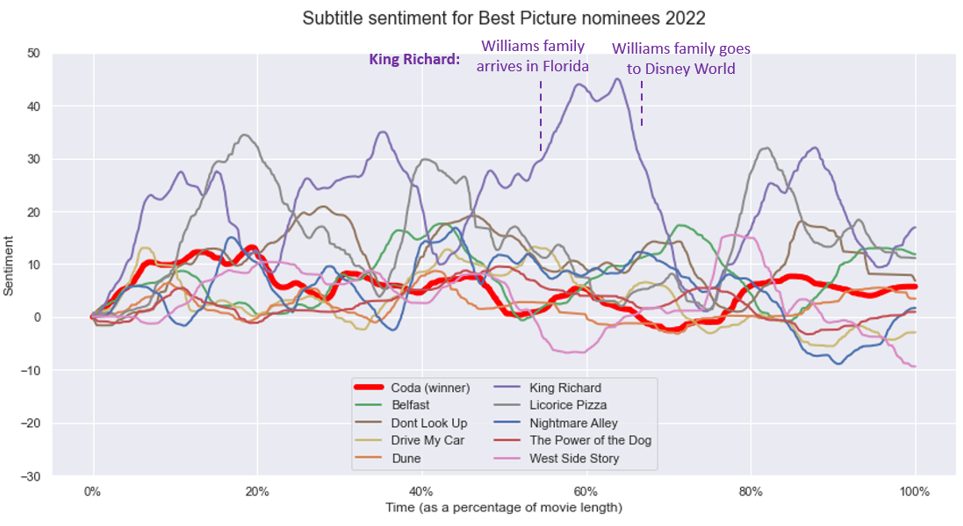
One of the biggest films of the year was King Richard, which is also one with the most positive sentiment of all the Best Picture nominees. The huge surge in positive sentiment intensity comes as the Williams family arrives in Florida and, weirdly enough, the downturn happens after the family goes to Disney World. Not the way around I’d expect… On closer inspection, it seems to be caused by some fast-talking celebration and plenty of subtitle insertions of ‘[laughing]’ and ‘[cheering]’ picked up by the model. Or maybe there’s just something really great about Florida (that isn’t Disney…).
Breathless tension
One of the key skills of data analysis is understanding the limitations of your models, your data and your analysis. While the method chosen has proven to reveal many interesting points in Oscar movies, there is a key weakness that some of you might have already considered.
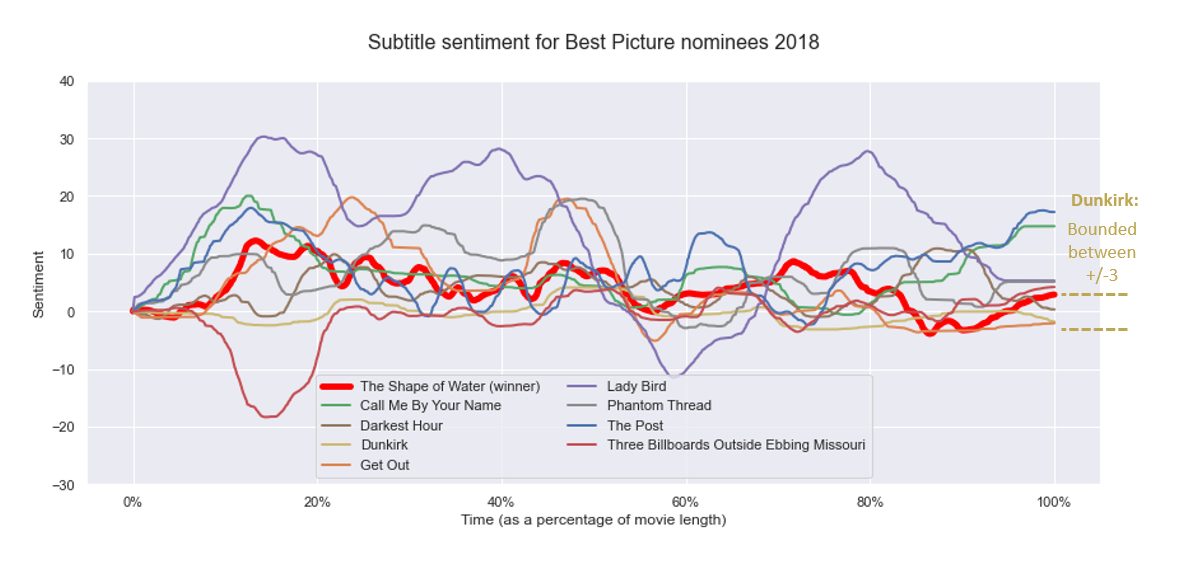
A movie full of spectacle, with countless moments of intense fear and celebration, has somehow managed to evade the model’s ability to pick up any moments at all. For those who have watched Dunkirk, it may not come as a surprise, because the movie manages to convey all this emotion through visuals and music, with very little dialogue at all. Due to this, the 10-minute rolling window often gives the VADER model very little to work with and so the wordless tension goes unnoticed in our analysis.
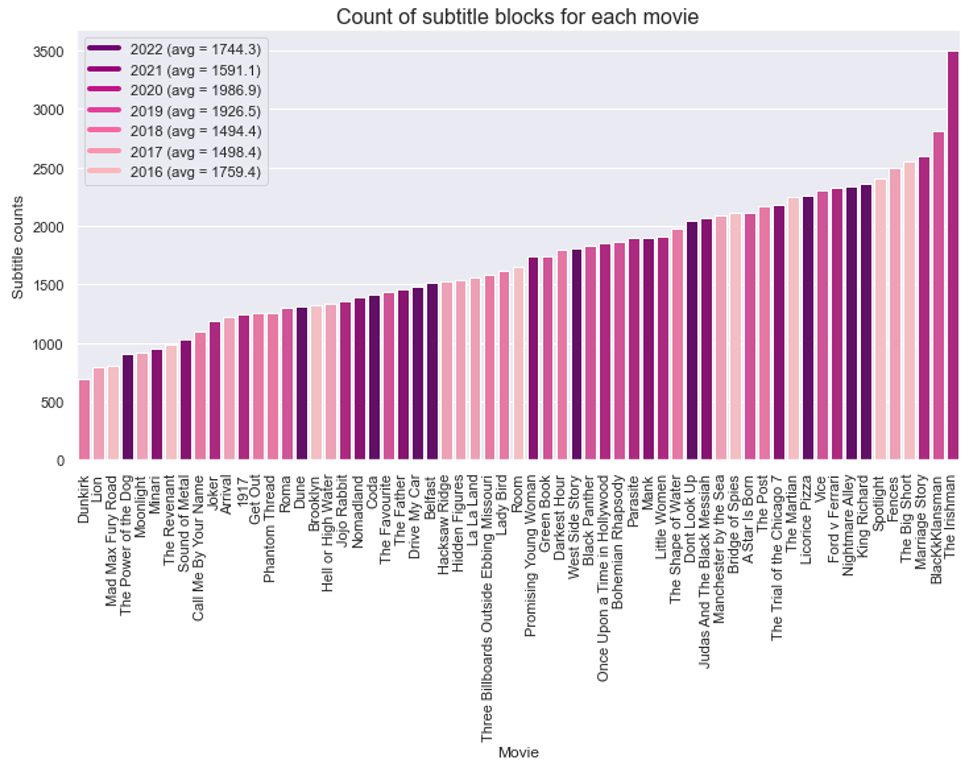
The disadvantage Dunkirk has can be seen very clearly when all of the movie dialogue lengths are laid out together. The fact that we knew to investigate further because we’d seen Dunkirk leaves us a key lesson around the importance of subject matter expertise when analysing data. And also the lesson that maybe Scorsese’s 3.5-hour marathon of a movie had 20,000 words too many.
Best in class
Some more interesting results can emerge when you aggregate many movies into specific classes such as ‘Oscars year’ or ‘Oscars category’.
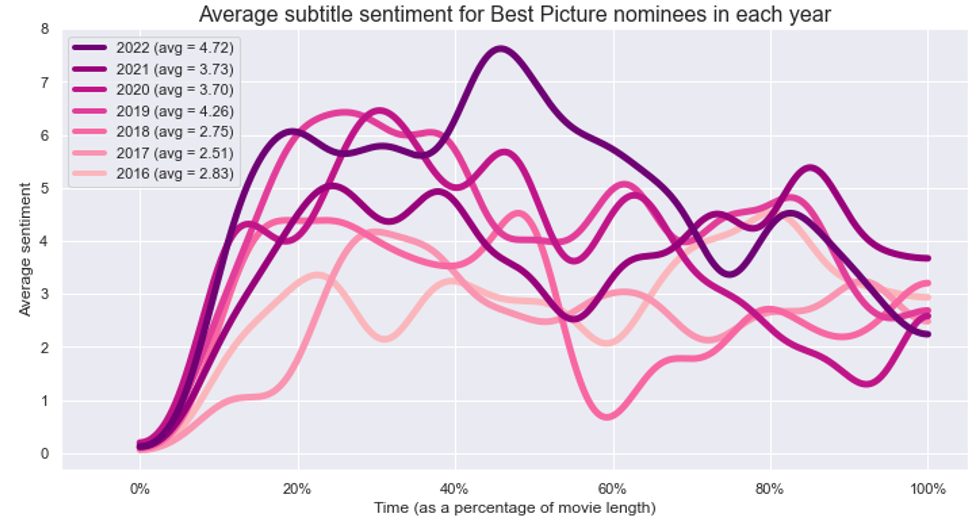
It looks like the Oscars have gradually been including a greater number of movies with positive sentiment. Does this represent an improving disposition of the Academy? Have top-tier screenwriters escalated sentiment as they noticed nominations going to the most positive dialogue? Or is this a reflection of humanity yearning for happier movies, needing an escape from the hopeless reality of a perpetually deteriorating world? Or is it just chance?
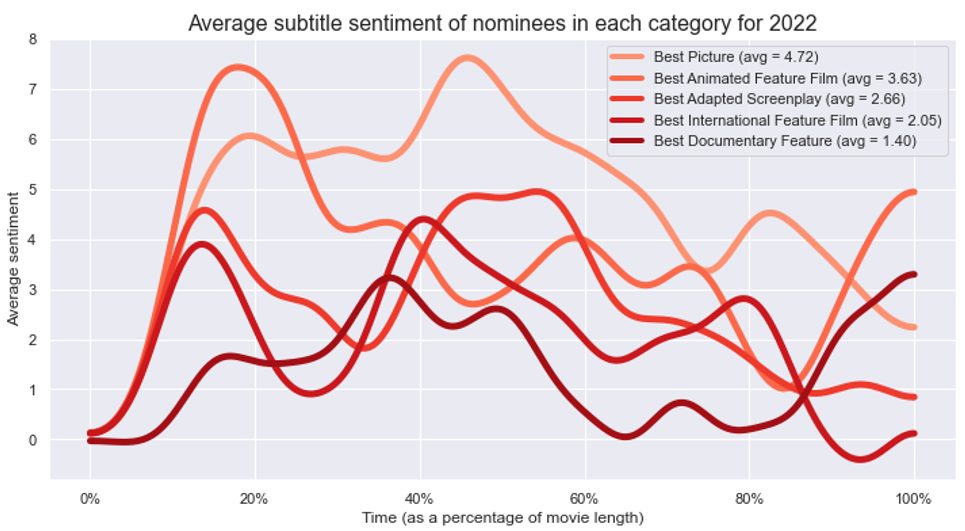
It’s surprising to see Best Picture still above the pack, ahead of even the Animated Feature Film category which this year includes three Disney movies (Encanto, Luca and Raya and the Last Dragon). Also notable is that international films haven’t reached the same lofty dialogue sentiment heights as their Hollywood counterparts, and the best documentaries follow the perception using more serious and more factual language than other categories.
But I think we’ve seen enough now from movie subtitles… it’s time to see what everyone else has to say.
The People vs The Critics
It’s been said that “any fool can criticise”. Well, let’s see how much the esteemed critics of Rotten Tomatoes agree with the average fool!
Each review on Rotten Tomatoes is binary (fresh or rotten) and the overall ‘rating’ represents the percentage of people who gave the movie a positive review.
- The ‘tomatometer rating’ is comprised of writers, bloggers, podcasters and publications approved by Rotten Tomatoes as embodying the key values of dedication and insight.
- The ‘audience rating’ is comprised of anyone bothered to make a free account.
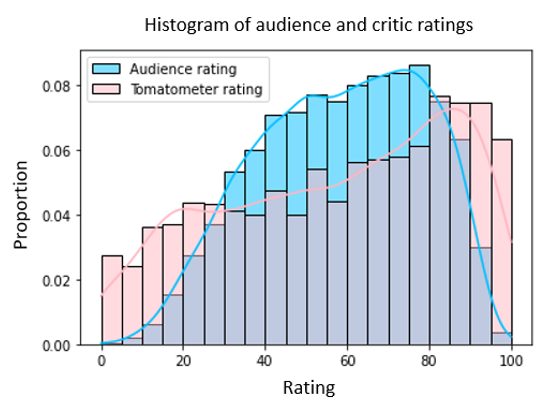
Looking at the histogram of ratings for movies from 2000 to 2017, the audience ratings tend to follow a nice almost bell curve, with the majority of movies getting a rating between 20% and 90%, and very few movies receiving a very high or very low rating. By contrast, the tomatometer ratings are quite uniformly spread across all rating levels.
Perhaps now is a good time to point out that if every rating on the site was decided by coinflip, regardless of movie quality, the resulting shape would be a bell curve.
The People vs The Critics vs The Academy
Overall, there seems to be quite a lot of consensus between audiences, critics and the Academy with the clear peak of each histogram sitting around the 80% to 90% rating. One notable exception is the 2007 film starring Eddie Murphy and Eddie Murphy called Norbit. This 9% tomatometer film has an Oscar nomination for ‘best achievement in makeup’, but unfortunately for Eddie, it lost out to La Vie En Rose, which had three fewer characters played by Eddie Murphy.
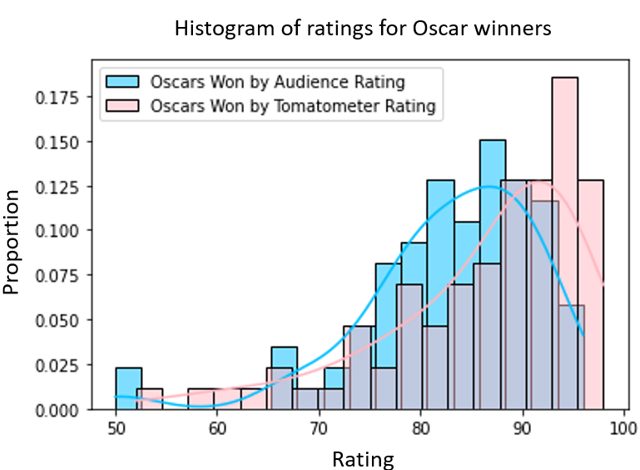
It’s when we get to the Oscar winners that we can really see the alignment between tomatometer and the Academy. This correlation is made possible by the higher number of 90+ ratings given out by critics. However, one thing to call out is that the audience and tomatometer ratings could be affected by Oscars results. Perhaps this means the tomatometer critics are just chasing clout by increasing their ratings of Oscar winners post hoc?
And with that baseless slander, we must bring this article to a close. As always, thanks for coming on this analytics journey with us! It’s been fun crunching data and talking movies. Reach out with any feedback or ideas on topics you want us to cover next.
CPD: Actuaries Institute Members can claim two CPD points for every hour of reading articles on Actuaries Digital.