Actuaries Digital
The peer-reviewed platform where actuaries share evidence-based perspectives on the financial, social and technological issues of today and tomorrow.
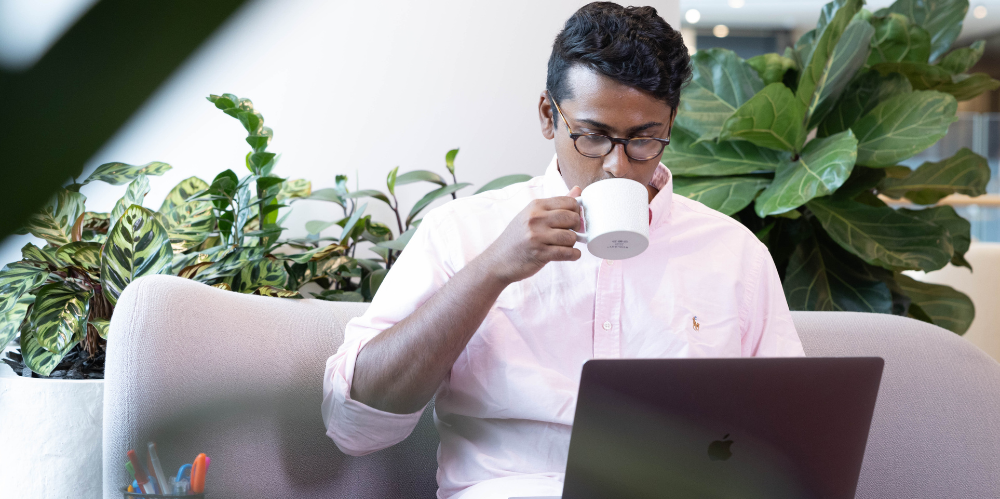
This month in Actuaries Digital
COVID-19 Deaths the Key Driver for 2024 Excess Mortality to November
In their latest article, the Mortality Working Group shares excess mortality analysis for the first 11 months of 2024. For the first 11 months of 2024, against a baseline that includes anticipated COVID-19 deaths, total mortality was 1% higher than predicted, which is just within the 95% confidence interval. There have also been five deaths from COVID-19 for every death from influenza. Explore the statistically significant outcomes that contributed to this result.
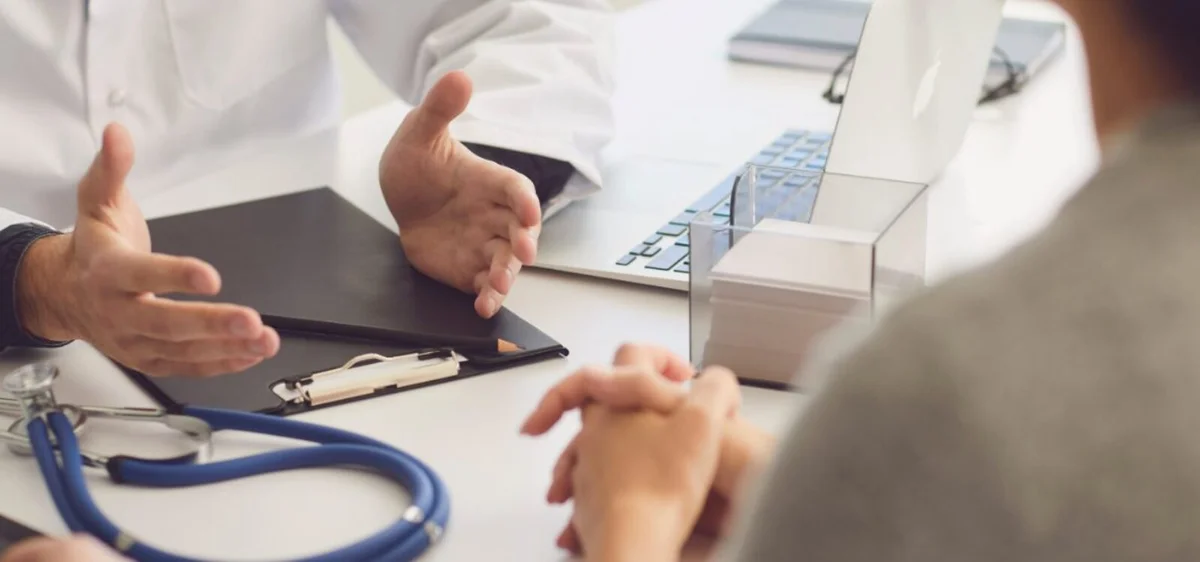
Trending topics
Unmasking Greenwashing with AI
As sustainability becomes a central focus in corporate strategy, businesses are eager to showcase their environmental credentials to appeal to investors, consumers and regulators. But not all that glitters is green. The rise of greenwashing – the exaggeration or fabrication of eco-friendly practices – has become a significant challenge, eroding trust and distorting critical data that actuaries rely on for decision-making.
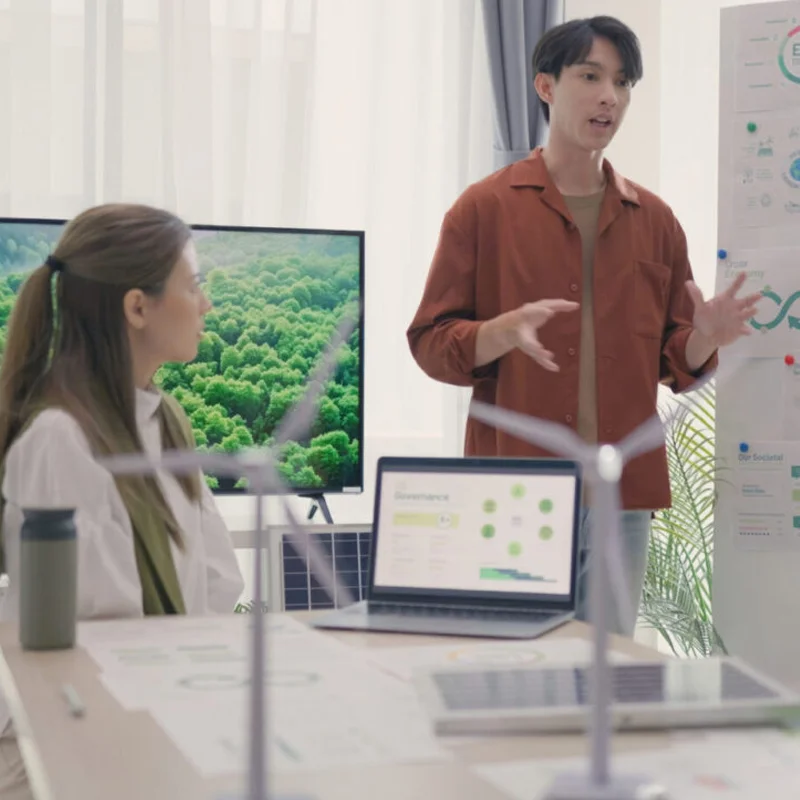
In conversation
Podcasts and videos
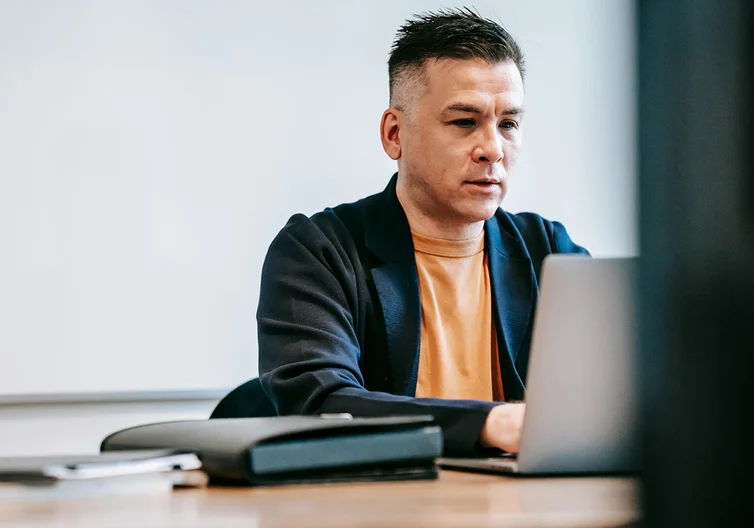
Meet the Actuaries Digital editorial team
The informative and inspiring articles published on Actuaries Digital are overseen by our editorial team, subject matter experts within our membership who dedicate their time and expertise to bring actuarial insight to current and emerging topics and challenges.
Lead the conversation. Contribute to Actuaries Digital.
We’re always looking for new research, fresh perspectives and candid conversations. Share your ideas with our editorial team today and you could be published in Actuaries Digital.
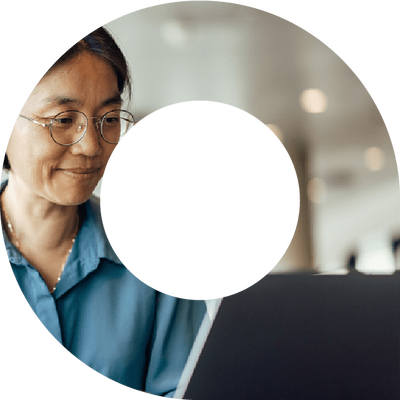