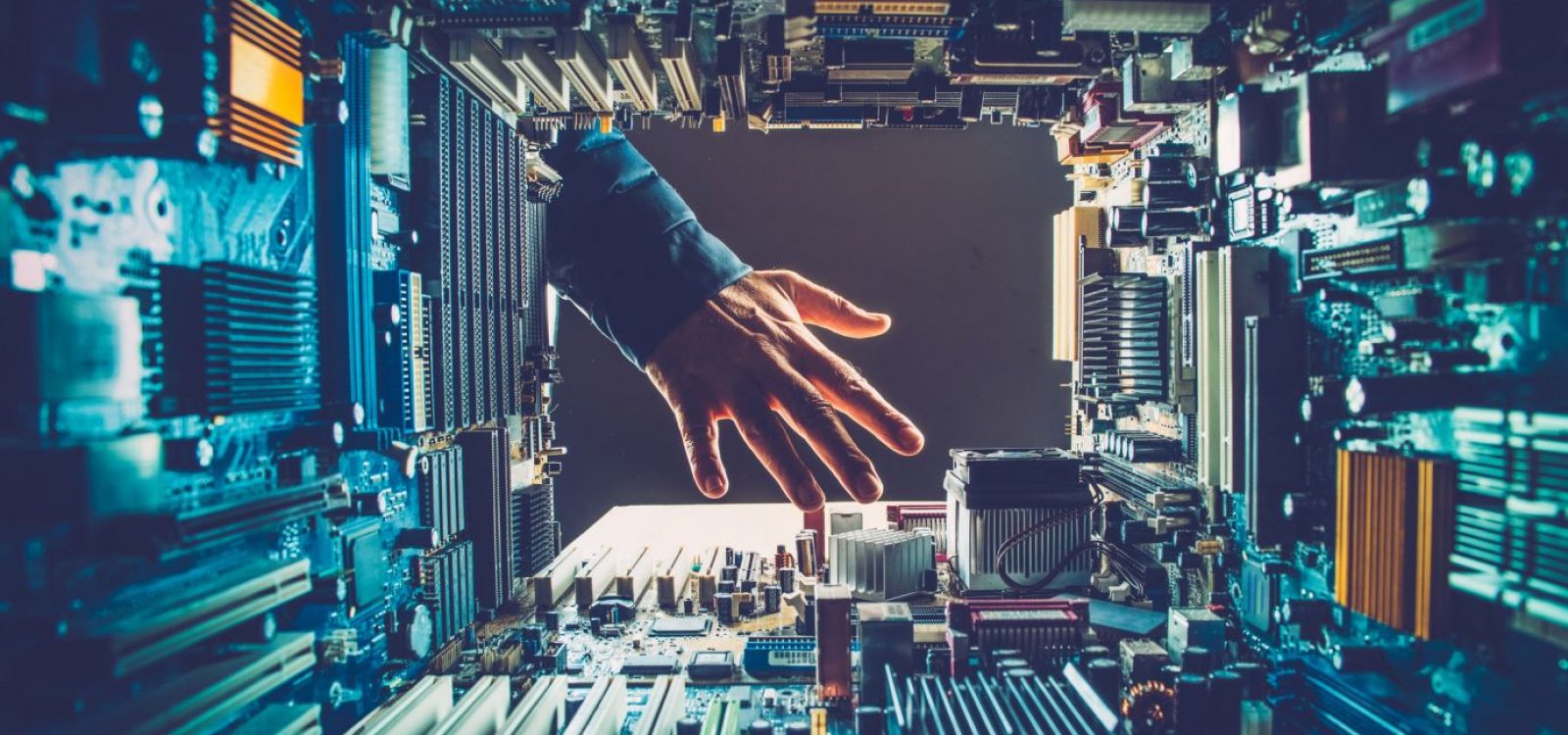
Ethical AI
Hugh looks at the EU’s recent guidelines for ethical AI.
As artificial intelligence (AI) becomes cheaper and easier to build and apply, it is natural that more attention has been given to the ethical issues surrounding AI.
Some examples are obvious – if a prediction model can be made more accurate (e.g. an insurance premium) using variables such as race, is it ethical to do so? However, there are other types of ethical issues, some subtle, that can arise too.
One interesting contribution to this topic is the EU’s “Ethics Guidelines for Trustworthy AI”, released in April 2019 and put together by a European group of AI experts. By expanding to the term ‘trustworthy’ the guidelines also include recognition that AI should also be lawful and robust, in addition to ethical.
The most practical part of the document is a toolkit of things to consider across seven areas that speak to different aspects of AI risk.
Taken from: Ethics guidelines for trustworthy AI |
For those interested, it’s worth a read, but some thoughts I had on the way through:
- Human Agency (#1) is a challenge for prediction engines. Many models produce a recommended course of action but won’t always contextualise choice for people making the decision and may lead people to trust a computer more than a human recommendation; in medicine for example, a treatment option often needs significant amounts of context that allow informed decision making. It also reminded me of David Wilheim’s presentation from the 2017 IDSS where car insurance repairer recommendations had to be both useful but promote choice and agency.
- Resilience to attack (#2) may range from serious hacking attacks (like hacking a model) through to gaming (e.g. a user might fiddle with inputs in an insurance rating engine to find cheap rates attached to unusual configurations). With cutting edge vision recognition systems able to be fooled by stickers, it’s a timely reminder than not all users of a system will do so in good faith.
- Transparency (#3) is an area that has developed significantly over the past decade. While models have grown more complex, there are a variety of ways to unpack a model, and see why a particular output is being produced for a certain set of inputs.
- Fairness (#4) is also an important topic, albeit more subjective. The first challenge is even defining it, which was well-covered in this paper by Chris Dolman and Dimitri Semenovich at the recent Actuaries’ Summit.
- Environmental impacts (#6) are worth considering, as the demand for computer processing time increases with the complexity of some algorithms, or the popularity of the product. For example, the Bitcoin network now requires the same amount of electricity as a small to medium sized country like Ireland, for a payments network that has significantly less throughput than other networks like Visa or Mastercard. Much of this mining is done in China, where about half the power is generated from coal. While not strictly AI, it’s easy to believe that the development of currencies like Bitcoin did not have environmental concerns front and centre.
More generally, it’s easy to see that some high-profile uses of AI will have to embrace more formal governance structures along the lines of the EU guidelines; executives, regulators and consumers will all have questions about computer models that need to be answerable, and AI-based decisions need to be defendable. This requires a good mix of technical skills, business understanding and concern for the public good; perhaps another opportunity for actuaries?
CPD: Actuaries Institute Members can claim two CPD points for every hour of reading articles on Actuaries Digital.